The Uptime Wind Energy Podcast
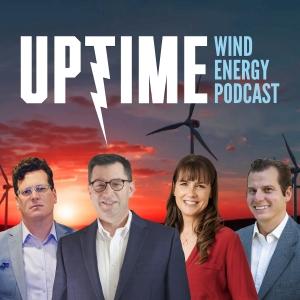
WindESCo Swarm System Steers Wind Farms to Higher Power
WindESCo’s Intelligent Swarm system maximizes wind farm output with revolutionary wake steering technology. VP of Products, Danian Zheng, and Director of Software Engineering, Pete Bachant, share their success story at the Milford I&II Wind Farms in Utah with Joel and Allen.
WindESCo – https://www.windesco.com
Pardalote Consulting at https://www.pardaloteconsulting.com
Wind Power Lab – https://windpowerlab.com
Weather Guard Lightning Tech – www.weatherguardwind.com
Intelstor – https://www.intelstor.com
Sign up now for Uptime Tech News, our weekly email update on all things wind technology. This episode is sponsored by Weather Guard Lightning Tech. Learn more about Weather Guard’s StrikeTape Wind Turbine LPS retrofit. Follow the show on Facebook, YouTube, Twitter, Linkedin and visit Weather Guard on the web. And subscribe to Rosemary Barnes’ YouTube channel here. Have a question we can answer on the show? Email us!
WindESCo
Allen Hall: Welcome to an exciting bonus episode of the Uptime Wind Energy Podcast, where we explore the latest innovations in the wind industry. I’m your host Allen Hall, president of Weather Guard Lightning Tech, along with my co-host Joel Saxum of Wind Power Lab. In this episode, we’ll be discussing the WindESCo Swarm System, a proprietary software platform designed to optimize the performance of wind turbine farms.
The Swarm system uses advanced analytics and machine learning algorithms to provide real-time data analysis and farm optimization, helping the wind industry operators and owners maximize the productivity and profitability of their wind farms. We’ll take an in-depth look at how the Swarm system works, is key features and benefits and real-world examples of how it’s being used to improve windfarm performance.
Joining us today to discuss the WinCo Swarm system is Danian Zheng. VP of products at WindESCo and Pete Bachant, Director of Software Engineering whether you’re a wind energy operator, investor, or just simply interested in the future of clean energy, this episode is for you. Danian and Pete, welcome to the program.
Pete Bachant: Thank you, Allen. Thank you, Joel. Thanks for having us.
Allen Hall: So today we, we see a lot of operators that are really struggling to maintain a profitable farm. They’re being squeezed from every possible side. And, WindESCo, you guys have been around since 2014 and have worked with a number of operators. What are you hearing from operators today?
Danian Zheng: So the biggest thing we hear is the, without PTC, a lot of people cannot even get profit at all, especially for the older farms. And then the income of those IPPs we call them, are really under pressure because of the High cost of the turbine side and the, and the maintenance side, and also high cost from the weight loss from the site.
Allen Hall: Wow. And, and if we don’t do anything to correct this, what happens? Do some of these operations eventually shut down because they’re just losing money? Absolutely.
Danian Zheng: You, you can see farms, they’re really only getting PTC as their margin out of the revenue and the day, typically after 10 or 12 years when they lose their PTC or the production tax credit.
Economic money, then you have zombies, sides just running there, nobody wants. Wow.
Allen Hall: Okay. So our discussion today is really in important then because the Swarm system helps bring some power back into their pocketbooks. And I, I, I. I wanna understand exactly what Swarm is. I’ve read a lot about the, the trade study with the Milford Wind Farms in Utah, which was fantastic by the way.
But I, I need a little more information. Like, h how does this system work? What does it do? How do operators implement it? Can you give us a, a brief description of
Danian Zheng: that? Definitely. I’ll try first and then I’ll leave some of the more high end stuff to Pete for Pete to us also. So it’s very high level.
The Swarm system is an intelligent farm level control system. One thing we notice is every farm got a lot of losses, but each turbine is optimized to work on its own best without knowing the performance of the other turbines around it. So you can have best local performance on this turbine, but it can generate enough wake.
To downstream turbine. So the next turbine is working at much lower level. So how to avoid that is by control all the turbines together. So that’s one thing we, people call it weak mitigation. And then there are other things we can look at the whole side globally. To see if turbines can work based on the overall, when the flow going through the whole turbine itself, the local ais and the local impact of turbine.
And then there are other things if we can introduce predictive control to that based on the turbines working up upstream and then the downstream to run, get time to adjust it for the best production. So if we can combine all of that together on the IOT based farm level control network. That’s swarm.
And then of course, this system cannot work with the best of the brain. That’s what controls on top of that. And I will leave that to Pete or really designer of
Pete Bachant: that brain. Yeah. So basically the reason swarm is necessary because as, as Danen said, turbines were always designed with. Just local control in mind.
They’re, they’re selfish. They just, they do their own thing. There is not some high level master controller that, that looks at the entire picture and tries to optimize the farm as a unit, which is actually really what you care about. You care about the farm producing as much power, not the individuals. So the way our system works is, is it takes that perspective of a centralized controller collecting data from every single turbine.
And kind of modeling that and trying to understand what is happening at every incident and time. Try to understand where are the different wakes and predict. If we were to change the position of this turbine or that turbine, what would happen to the power? What would the consequences be of, of turning these turbines different ways?
If we can predict that quickly enough, we can then find the optimum positions of all the turbines and we can send out those signals and actuate it and, you know, mitigate some of these weight losses, mitigate some of the inaccuracies in estimating the wind direction at different turbines throughout the plant.
And ultimately make up for that kind of, that deficiency in initial design of only thinking about local control. So we’re retrofitting on and we’re making up for that problem. Well, the one that, you
Joel Saxum: know, from our, our talks off air, the one big thing that comes into my mind here, you know, I’m always, I’m always playing devil’s advocate when I see a new solution, right?
Cause I, so I want to ask the questions that the asset owners might ask. First one that comes to mind, cybersecurity. Right. If you’re connecting an iot device and you’re connecting a whole wind farm, how are you guys handling that aspect of things and making sure that the asset owner or at the farm level is safeguarded from any kinds of, you know, cause I know that FERC and stuff had, they have standards that they must meet to make sure that the grid isn’t accessible from, I will say outside stakeholders.
But how are you guys handling
Danian Zheng: that? Definitely. So this is, Always the number one question coming from customers, IT department, and the overall leadership. So the, we treat that very, very seriously and we have good cybersecurity in the whole design. So fundamentally, the biggest part to keep fiber cybersecurity work for product is we keep the farm level control onsite within the premises of the site.
So there is no external access into the site that’s beyond the typical state of art security access for, let’s say, to update SCADA server like everybody does daily or monthly. So we don’t have any. Let’s say cloud-based, real-time intervention from outside, which is something if you talk about everybody gonna run as fast away from you in this world.
And also we design our software to be and our data flow to be able to Really confirmed to the design guidelines coming out of the from organization side, the is O 27,001 and from the design side, the I E C 6 2 4 4 3 guidelines we’re not exactly there yet, but I know everybody else is in the same water and we’re all swimming toward the
Pete Bachant: same direction.
I’ll add that from an architecture standpoint, our system is cloud connected because it, it leverages the cloud to. Monitor data, perform analytics on the system, offline, retune models, check, make sure the models are performing like they should. Things that you wouldn’t really want to do on-prem cuz you’d have to store months and months of high frequency data.
So we’re leveraging the cloud, but we’re only interacting with it outbound connections. And these are all encrypted and use authentication and authorization. So nothing. There’s no inbound traffic allowed into our controller. Basically, Pete, there’s,
Allen Hall: there’s algorithms behind this that determine the optimized position of the wind tournaments, and it must be, it must vary by wind speed and probably a number of other factors.
How are those algorithms developed and are those algorithms site specific? Yeah,
Pete Bachant: so the way that we do it, I mean, you can look at some other, I guess, competing technologies out there in academia and, and different products available And I think the, the kind of most typical way that, that someone will do it is use a lookup table.
Like you take a farm, you simulate it in a bunch of different wind directions, wind speeds, maybe turbulence, intensities, come up with a lookup table for what’s the optimum position of each turbine to do wake steering in this case. And the problem with that is that it’s really. It’s a very multi-dimensional problem.
So if you want to add, so we’ve got three dimensions. There we go. Wind speed, wind direction, turbulence, intensity. Okay. What if we want to take into account the fact that different turbines are gonna be offline for different reasons, different turbines might be derated. You just added two more dimensions and these things compound geometrically, so your lookup table would have to be huge.
So anyway, we use a model-based controller. We have a, a model that can predict the flow through the farm very rapidly in real time in our controller. And we leverage open source tools such as Nres, Floris on top of which we’ve put this wrapper to achieve what you’re saying, like the, the specifics of the site.
I mean, it’s. You could take this tool, Floris, right? And you can say, okay, the wind speed is X, the wind direction is Y, the TI is Z. And you get a prediction for your, for your site. But the process of taking your SCADA data or any other sort of measurements and translating it into the appropriate wind speed direction in ti.
Takes a lot of work. So that’s kind of, I guess like the secret sauce that we put on top is how do you estimate the inputs properly into these simplified engineering wake models to make it match the behavior of the site and make it match over a long period of time. So we have lots and lots of historical data.
We take the model, we train it, we use data science tools to, to make sure that this thing is going to work in real time. We have our model trained. Deployed out to the site and we use it directly in the controller.
Joel Saxum: So with that being said there, there’s a process to it, right? So what is, if, if and I’m gonna go more to the commercial side.
If customer x, y, Z contacts ESCO and says, Hey guys, we’d like to know what swarm is and possibly put it on a wind farm from that is T zero. How long? Time-wise, are they until they’re seeing the benefits of the system, is it until you get it all implemented, the model’s trained up and everything’s working?
Is it two months, six months, a year? How long is that normally gonna take for a client to see the
Pete Bachant: results? Many times our clients can actually send us a, a good chunk of historical high frequency data and that that gives us a good running start. We can train models upon that. We can get a sense for how the farm works, how heterogeneous the flow is through the plant, things like that.
There is gonna be a little bit of a transition period when we actually put the swarm edges that we call them onto the turbines with their own specific sensors. The character of the wind speed and wind direction measurements changes slightly at that point in time. But we, we can hit the ground running pretty well start doing optimization from day zero.
And the nice thing about our process is that. The optimization may be not optimal at that point in time, but we’re continuously acquiring this operational data, sending it up to the cloud, and we can then retrain the model and reassess. Are we making these predictions properly? Are the wakes where we think they are at every given point in time?
And yeah, the model just improves and we’re thinking like the, the rule of thumb now is by the time you get to six months, as long as nothing crazy has changed about the sensors like, The system has learned how this is going to work and it’s gonna optimize the plant the best it can at about the six month mark.
Yeah.
Joel Saxum: So we’re saying like basically within a year, because everybody’s got a different, every wind farm is gonna have different weather pattern, right? Based, and it’s a yearly cycle. Contrast is, it’s not a sine wave, but we kind of know that the heavy wind season is this and the light wind season is this and the winter is this.
And so, If you can get it kind of trained within six months, basically six months, you’re starting to see a little bit of you know, a p increase in that, you know, that one to 5% range. And then as that year kind of closes out, you can start to see the real results of over the course of the year when the weather patterns change and what your traditional a e was.
You see those little bit of increases. So it sounds like. If a, if a client wanted to do it right and get going on it right now, now it’s busy season starting up, right? It’s spring in the us. They might be by fall making, making some
Pete Bachant: good gains. Yeah. That also highlights one of the, the benefits of doing it.
Like we call this a closed loop model, which means that you don’t just acquire six months of data, train the model. Make it static, it just sits there. We keep doing it. We’re, we’re continuously collecting the operational data. We send it back into this validation and calibration process. So yeah, at six months you’ve done a lot of learning.
To, to calibrate the model, to match the site behavior. But you keep learning over time. So if you get out to a year and things have changed, now we actually do see some sites that are very different. You know, one six month period to the next six month period. As you keep accumulating that data, you keep accumulating the learning in the model.
Allen Hall: What happens when your next door neighbor puts in a big wind farm? Does that really make the model have to readjust? Cause that’s what’s happening right now. We’re putting wind farms on top of wind farms on top of wind farms. Their wakes have to be changing yearly. Right. So you need an adaptable
Pete Bachant: model for that.
Yeah. I mean that would be a big step change, I guess, in how the model works because you think about that transfer function from like your inputs to the actual behavior of the plant. Something is gonna happen. Differently in that process, if all of a sudden you put another farm upstream, your measurements of wind speed at all, your different turbines are now different in character.
So the model’s going to have to learn that new behavior. I dunno, brings up an interesting question about like private property or something like that. Do you have the right to steal the incoming flow from your neighbor or something like that? Because it, I mean that, that’s like taking money that was flowing into their plant.
But
Danian Zheng: one point on top of that is you know, because. Pc, right? The model itself is not a static model based on, let’s say, just taking Floris and the plug some numbers, right? This is continuous learning. It reduces the impact from the up upflow changes right along time. We can learn and adapt
Allen Hall: to that. So I, I know there’s some hardware associated with the swarm system.
Can you walk us through a little bit what an operator would see in terms of hardware installation and sort of the timeframe to get it installed and what that means and what the, what the hardware does?
Danian Zheng: Definitely. So I actually browed to the podcast. Just look behind me. That’s the swarm Edge. Box, we call it internally.
Pete just mentioned earlier, it is iot device. We put on top of the nael of the turbine, typically connected to the turbine control system. And that is connected also to various site server, which is really just Just look like a scaler server in the server room. And remotely we connect is on the site business network.
And that’s how to draw earlier discussion about cybersecurity. We’re not on the turbine control network, we’re on the business network typically where the, you know, c m s system run, right? So we stay away from the most critical part safely. And this box, what it does is take the turbine realtime information.
Take the SCADA information, take our own sensor information altogether, and then take a command reference or command direction from the, we call the swarm server from the site level and drive the turbine to the position it should be at. And we’ve does that in a very G graceful way, which is OEM ag agnostic.
This box right now have about eight to 10 different interface built in already. And we can adapt to different turbines differently, depend on their communication scheme built in. And also it has a bypass system where there’s a watchdog looking if anything goes wrong, the swarm edge will take itself out of the turbine control system and the allow turbine to handle everything by its original design.
So you talk about the installation time. So this is really at a similar level of complexity of installing, let’s say a C M S control node up power takes about half day for trend technician to give us through. Takes a, another, maybe a couple weeks for the whole site to be commissioned and connected together.
And then we can hand it over to Pete to start really we call
Joel Saxum: swarming. So this is when we’ve started seeing the, you know, the swarm system where any, any kind of wake steering when I, you know, following Flores and following some other things that en R was doing. Coming from Wind Power Lab, we’re always worried about fatigue loads on blades.
What’s gonna happen to these things in the future? Could this affect. Bearings, what, you know, what are the, what are the outside effects that could happen from the system? So the, you know, when we talked in the past, I, I, I think we, you guys had talked about some d and v certifications and stuff for this, for the the swarm system.
So can you walk us through some of those things that will put them, the, our listeners, you know, their mind at ease when it comes to you know, external factors that might. Affect the turbine system
Danian Zheng: itself? Absolutely. So we, we really treat the design of the safety and the turbine life site very seriously.
And we started very early on realizing the weak steering and the general y control intervention we’re taking. Well in introduce additional. Misalignment and that will have a possible negative impact to the turbine loads especially for the upstream weak steering turbines we call. Right. So what we can do with that is, let’s see how much it is and compare that to the turbines original.
Loads condition and see how much delta we’re introducing in and how that impacts the loads. So what we did is we actually went through two separate effort. The first effort is for the Milford study, not study, sorry, installation. Now it’s a commercial project. We actually went through a full onsite study for the site specific loading and the safety operations with D M V G L.
That’s back in 2021. That’s what we did before. We are actually allowed to install in scale on at the site. Right. So that’s, so through that study we figured actually, although we are adding some loads to that, but the level is so low, we’re really at, you know, 1% less than 2%. That level compared to the original loads on the fatigue side, right?
On the extreme side, were not even the problem because most of those turbines has a 40 degree to 45 degree yellow misalignment. Extreme loading. Certification right? Already from anybody likely or, or ul. So, so it’s really mostly a fatigue problem coming from the upstream turbines. And by the way, all the downstream turbines get much less turbulence now, right?
So they actually have better fatigue load conditions. Now, for those upstream turbines, we. Based on the meal first study, we actually build a method to evaluate that. And we have worked through the whole last year with UL to bring that method to a type certification kind equivalent to the wind turbine pipes certification where we look into, right, we look into the, the functional and the design safety of the turbine.
As a whole, right after adding our device, we look into the lows condition. We look into the site testing, and we have to be qualified by UL based on our site testing results. After that, we have three UL report covering all of that. We have a UL approved a method to calculate the loads, and UL can actually audit and check on that result.
And based on that method and result, we can really recommend to the customer and the tumor or controller to make sure the site is under the low condition that will not negatively impact the life. So that’s how we get it.
Joel Saxum: That’s fantastic. So anybody that’s got a little bit of a, you know, like a hair up on the back of the neck, like, oh, could this affect, you know, the, the loads on the turbines, you’ve got the backup, you’ve got the data, you’re, you could be send it over to ’em in a zip file and ease their mind.
Danian Zheng: Absolutely. And the, that’s a great point, Joel. Right. So this is the front end prebuilt, right? We call it in the wind industry post build, actually piece. One way, remember he talked about the one way data collection in the cloud. Right, and he will be building what we call a audit trail, right? A track record of how we’re controlling each turbine and will saved somewhere, right?
If something goes wrong in the future. We can provide evidence to our I P P customer and allow them to work with OEM to do the root cause analysis based on our data to most likely to say we’re not a corporate, maybe something else is at work there. So that’s the other part post build. Yeah,
Joel Saxum: more data’s always good.
Pete Bachant: We’re not just doing simulation. We actually have blade load measurements from the system in operation. And I think you’ve seen this in, in the Milford case study. So we’re actually tying this back to, to reality too. And, and the loads. We’ve, as Danen said, we haven’t been collecting data for, you know, 20 years.
We’ve, we’ve got a decent amount of operational time now, but, but the loads are in line with what we simulate, so definitely not scary.
Danian Zheng: Yeah. And we have this system called, we. Protect from is a legacy product that actually have sensors on the turbine collect data to marry that. We have
Allen Hall: 165 turbines in operation with the swarm system.
That’s, that’s pretty impressive. And I, I know it is taking a number of years to, to get to that point cuz you need to do those. DM V G L checks, those UL checks. Those are really important to get the system certified and validated so that it can be used worldwide once they implement the swarm system.
What kind of a e p improvements are they seeing from, like, what are you actually measuring from the field right now?
Pete Bachant: Yeah, so, so far our measurements have been restricted, like to only a couple turbines. With those couple turbines we’ve seen, I forget the exact numbers, but we’re seeing. Something like 2% a e p I think we, we saw 1% being lost on average by the upstream turbine when we’re doing the wake steering and you get 3% gain on the downstream turbine.
So kind of balances out to about two. We expect on the whole one to 2% gains from wake steering alone. When we extrapolate out to the entire site and get the model tuned and everything, all commissioned and operational. Swarm does have some additional features, however, for more a e p gain. So our other product, fine fix measure, one of the main features of that, it’s it’s turbine level analytics and, and finding performance opportunities for the turbines.
Static yamas alignment. If a turbine is not on average, pointed in the direction where it’s gonna get the most power. In a situation where it’s not useful to do wake steering, we have the analytics to find that optimum angle in the cloud. And now once we add swarm, we can automatically deploy that optimization to the plant.
So it’s kind of a nice follow on feature in, in some cases, like if you want to correct static yamas alignment. It could take you going out to the turbine controller itself, you know, plugging a, a laptop in and adjusting the parameter, or even having to mechanically rotate the sensor on the top of the neel if you have a swarm system, well, we’re calculating what your yamas lineman is.
We’re actuating it directly. That’s probably another 1% on average in, in some cases, and that should be mostly additive because it’s, it’s. Applicable in the times when Wake steering isn’t necessary to do. And we have some other things we’re looking at as well. Like Danen was talking about the the ability to do some predictive ya or dynamic y make the turbine move more quickly to where we think the wind is going.
That’s a little bit more in development needs, definitely some more tuning, but on the whole, we’re hoping for. From Swarm as a whole 3% at Milford,
Allen Hall: that, that’s actually a really huge number. Yeah, you’d
Joel Saxum: be surprised at how many turbines in the global fleet have that static ya misalignment. We see it on a, a lot of like we would look at some SCADA data for some root cause analysis, and it’s always like, I would say the majority of them are not quite right.
Pete Bachant: Fine. Fix measure is just, you know, analyzing high frequency skated data
Joel Saxum: there. I mean, if, if someone’s not ready for the swarm system yet, that’s a good icebreaker to get. Working with ESCO on getting some extra performance out of your wind turbine farm as well.
Pete Bachant: That brings up an important new.
Feature that gets unlocked when you have a swarm system because this is also a generalized iot platform. We add some more sensors in, we can do additional analytics and find. Additional opportunities because we’ve got more sensors. So I don’t know, Danny, if you wanna go into some examples of the things we’re, we’re looking at developing now that we have more
Danian Zheng: data.
Definitely. So if you look at, again, referring to that beautiful box behind me, right? There is a full network control system inside there with a very capable plc which have at least quad core, right? If we want, we can do even more cores in there. That capability is really just half running right now, right?
With this system. Now, if we add additional sensors, We can do blade monitoring, we can do power foundation monitoring, we can do give us vibration, some of the CM, cms. All those things can go into this iot platform based on what app you deploy there and what sensor you deploy there. And one big thing, one was back in GE doing wind turbine design is the cost on the wind turbine for any equipment to get on the wind turbine.
There is a cost. Threshold you have to go through, right? And this computer, typically this device is the higher threshold part. Once you have this on the turbine, adding sensors are relatively low cost. Adding apps are. Very low cost. So there, this opened up the door of a lot other applications on that.
So we’re looking into a couple reliability related applications already with partners and all those things gonna come in the
Allen Hall: future. There’s a lot going on at ESCO at the moment. The swarm system, the fine fix measure and new things on the block. I know you can check out ESCO on LinkedIn too. They have a nice LinkedIn page and you can follow Danen and Pete.
On LinkedIn. Hey, Danian and Pete. Thank you so much for being on the podcast. I’ve learned a tremendous amount. I know Joel has also, and we’ll keep an eye on for more swarm systems in the, in the future.