Blogs, Whitepapers, News & Customer Success Stories from Blue Ridge
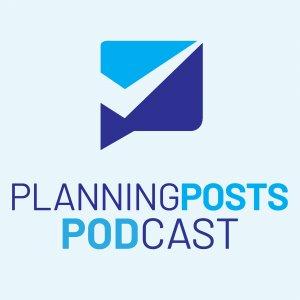
Driving Value with AI in Supply Chain Planning
In this episode, PlanningPosts talks with Rod Daugherty, VP of Product Strategy at Blue Ridge, about how AI and machine learning can be integrated into supply chain planning to drive value. Episode 7 Show Notes Todd: Let’s kick things off by telling us what is AI? And how has it made its way into the supply chain? Rod: You hear a lot these days in the market about AI. Machine Learning techniques are a subset of AI sometimes called applied AI. The challenge for us is finding ways we can use AI in supply chain planning to enhance business results. AI is prevalent in the consumer side of the market, and we all interact with artificial intelligence programs of some kind. For most of us, it is natural-language processing like Siri, Alexa or using spoken commands to make calls while in your car, for example. We have found there is an opportunity to take the marriage of the most optimal demand planning and inventory optimization models to more intelligent levels that help organizations achieve better profitably and efficiency in your inventory investment. Todd: Let’s set the stage a bit on artificial intelligence. Adding sophistication adds complexity, right? Rod: Well to some degree, yes. But in all of our design and development efforts, the goal is to make the user experience as efficient as possible, while enabling the solution to do the hard optimization work. The same philosophy applies with AI. The software should do all the heavy lifting and present actionable recommendations to the user to improve the experience and make them more efficient at their job. Artificial Intelligence must add real, practical value. Todd: Ok so how is AI different from traditional rules-based computer software processing? Rod: Traditional rules-based technology expects input that runs through predefined parameters and models and produces an answer. In AI, the input is still data, but is also the desired objective. Along with getting to the answer or recommendation, AI / Machine Learning creates or evolves a new model based on its own feedback or learning loop. It is not just selecting a model based on rules. AI models score themselves and continuously make corrections to arrive at the optimal recommendation. Todd: Got it. So let’s talk use cases. What are some areas where AI can add value in Supply Chain Planning? Rod: We are now moving beyond the traditional demand planning methods that rely exclusively on demand history and beginning to incorporate outside-in, third party data. An example of Applied AI that observes and learns is weather data. We are currently working on training models to understand and help predict how weather could impact sales for a distributor or retailer. We can: * Learn from observing user interaction with demand exceptions – detecting and monitoring – to automate & augment the human decision process * Learn from consumer behavior to drive targeted promotion and pricing recommendations – to anticipate and predict * Consumer search trends that lead to new product recommendations, which in turn improves the human decision process This requires Neural Networks that can deal with classifying very large data sets which can only be accommodated through cloud-native demand planning software. The app has dynamic access to as much processing power as required for a particular analysis, which can combine and blend disparate data sources. Todd: When we think AI, many of use thing about familiar AI apps like Cortana or Alexa. Could these be applied here? Rod: Well Todd, yes. These apps can translate spoken commands and questions into action. One possible application would be using AI/Natura...