Phantom Power
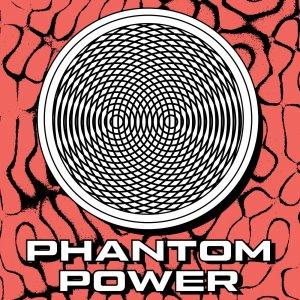
Are AI art and music really just noise? (Eryk Salvaggio)
In this episode, host Mack Hagood dives into the world of AI-generated music and art with digital artist and theorist Eryk Salvaggio. The conversation explores technical and philosophical aspects of AI art, its impact on culture, and the ‘age of noise’ it has ushered in. AI dissolves sounds and images into literal noise, subsequently reversing the process to create new “hypothetical” sounds and images. The kinds of cultural specificities that archivists struggle to preserve are stripped away when we treat human culture as data in this way.
Eryk also shares insights into his works like ‘Swim’ and ‘Sounds Like Music,’ which test AI’s limitations and forces the machine to reflect on itself in revealing ways. Finally, the episode contemplates how to find meaning and context in an overwhelming sea of information.
Eryk Salvaggio is a researcher and new media artist interested in the social and cultural impacts of artificial intelligence. His work explores the creative misuse of AI and the transformation of archives into datasets for AI training: a practice designed to expose ideologies of tech and to confront the gaps between datasets and the worlds they claim to represent. A blend of hacker, researcher, designer and artist, he has been published in academic journals, spoken at music and film festivals, and consulted on tech policy at the national level. He is a researcher on AI, art and education at the metaLab (at) Harvard University, the Emerging Technology Research Advisor to the Siegel Family Endowment, and a top contributor to Tech Policy Press. He holds an MSc in Media and Communications from the London School of Economics and an MSc in Applied Cybernetics from the Australian National University.
Works discussed in this podcast:
The Age of Noise (2024)
SWIM (2024): A meditation on training data, memory, and archives.
Sounds Like Music: Toward a Multi-Modal Media Theory of Gaussian Pop (2024)
How to Read an AI Image (2023)
You can learn more about Eryk Salvaggio at cyberneticforests.com
Learn more about Phantom Power at phantompod.org
Join our Patreon at patreon.com/phantompower
Transcription by Katelyn Phan
00:00 Introduction and Podcast News 03:24 Introducing Eryk Salvaggio, AI Artist and Theorist 05:33 Understanding the Information Age and Noise 09:14 The Diffusion Process and AI Bias 33:35 Ethics of AI and Data Curation 39:09 Exploring the Artwork ‘Swim’ 45:16 AI in Music: Platforms and Experiments 01:00:04 Embracing Noise and Context
TranscriptEryk Salvaggio: I think as consumers of the music generated by AI, that’s the thing that I want to think about is as a listener, what am I hearing and how do I listen like meaningfully to a piece of AI music that essentially has no meaning.
Introduction: This is Phantom Power.
Mack Hagood: Welcome to another episode of Phantom Power, the show where we dive deep into sound studies, acoustic ecology, sound art, experimental music, all things sonic. I’m Mack Hagood. Today we’re talking to the digital artist and theorist, Eryk Salvaggio. We’ll be diving into the question of what is AI art and AI music? And we’re going to attack this question on both the technical and the philosophical level.
We’re also going to talk about how to live in what Eryk calls, “the age of noise”. It’s a really interesting conversation, so stick around. But first I want to just go over a few quick show notes. For those of you listening in your podcast feed, you will have noticed that after something of a hiatus, We’re back.
I am looking forward to bringing you this podcast, once a month in 2025. We have a lot of fascinating interviews on tap next month. Journalist Liz Pelly will be with us to discuss her new book on Spotify. I could not be more excited about that. For those of you joining us on YouTube or maybe Spotify, you’ll notice that you can see me.
So it’s taken a lot of work, but we have officially jumped on the video podcast bandwagon. I think today’s episode is going to show the power of that, because we’re going to be talking not only about music, but also about video art made by AI. And it’s going to be helpful to actually see it with your eyes. But no worries to all of our dedicated audio listeners and visually impaired folks.
We’re going to be sure to describe anything relevant that’s seen on the screen. So audio or video, feel free to enjoy Phantom Power in the modality of your choice. And if you’re watching or listening for the first time, please do subscribe wherever you’re encountering this flow of waveforms and pixels.
And finally for longtime listeners who have been following along with my epic saga of trying to pivot from writing academic works to writing for the public, I’m thrilled to announce that I got a book deal. My next book will be coming out on Penguin Press. And for those of you who have been following along with this saga, you’ll know that I’ve done episodes and Patreon posts about how I found an agent, what it’s like to work with an agent, writing a proposal.
and so I’m going to have more bonus content in my Patreon feed where I talk about the final stages of how we crafted the proposal and shopped it to publishers and had meetings and had an auction and all that kind of stuff. So if you want the inside scoop. Just join our Patreon at patreon.com/phantompower.
Okay. Onto today’s guest. My guest today is Eryk Salvaggio. Eryk is a researcher and new media artist interested in the social and cultural impacts of artificial intelligence. His work explores the creative misuse of AI and the transformation of archives into datasets for AI training. Eryk is a researcher at the Meta Lab at Harvard.
He has advanced degrees in media communications, and applied cybernetics from the London School of Economics and the Australian National University. And you may know Salvaggio from his widely read newsletter on AI, Cybernetic Forests. I met Eryk last year at the Unsound Festival in Cracow, Poland, where we were both speaking and Eryk gave this dynamite performance lecture called the age of noise, which incorporated some of his video experiments with artificial intelligence.
And this talk just blew me away. I knew I wanted to bring him to you. So today we’re discussing how AI systems literally dissolve human culture, images, video. ,music, they dissolve them into noise and then use that noise as a starting point to create new objects that look and sound like cultural objects, yet lack human characteristics.
So welcome to the age of noise.
Here’s my interview with Eryk salvaggio All right, Eryk welcome to the show.
Eryk Salvaggio: Thanks so much. I’m really excited to be here.
Mack Hagood: So I had the pleasure hearing you speak at the Unsound Festival in Krakow. And I was just blown away by your talk, which concerned the role of noise in generative AI. And it also made a larger point about noise and contemporary digital life. And the central claim of that talk was that we have basically finished the information age and we’ve entered this, what you call, “age of noise”.
I think we’ll eventually make our way to AI and the age of noise, but I was thinking maybe we could start off by how would you characterize the information age? It’s certainly a term we’ve heard a lot, but how are you thinking about it? Say in the talk that, you gave.
Eryk Salvaggio: If you look at the early age of computing, if you look at the early age of communication, there was this belief and it’s not necessarily a wrong belief, that the more information we have access to, the more knowledge we have about the world and the more agency we have in the world, the more informed our decisions could be.
And so much of technology in that century, starting in the cybernetic era of the forties and the fifties even was around., “How do you get information? And make sense of that information?” And then when we started moving closer to the communication networks, it was more about , “How do you distribute this information so that everybody has access to this information?”
And all of it was around this idea that information is super valuable and that if we have information, we could become in a way, better people, better citizens.
And then with the internet. It becomes this weird mirror where everyone’s able to access information, but they’re also able to produce it. And the production of information is, measured and it’s weighed and it’s distributed by this sort of other worldly power that we’ve come to call the algorithm.
And so everything’s being sorted and we don’t necessarily have access to the information that we need to understand the world. Instead, we have information that is a mess, right? And it’s a fire hose. It’s overwhelming. And so my argument in the age of noise is that this information age piece that was just this access to information has become so overwhelming and so hard to process that it has become essentially noise.
Mack Hagood: Yeah. And I loved like there, there’s a point in the talk where you’re talking about the role of noise in the information age. And you’re basically talking about information theory and we should probably have a drinking game for this podcast at time. I mentioned Claude Shannon, cause
I always mentioned Claude Shannon. You talk about, how noise was this thing that sort of crept into circuits and crept into the
channel and that noise was this residual energy of the big bang.
and our task was to remove any traces of noise from our phone calls. And, then we get to this point where the information age has given us so much high quality signal, so to speak, that in itself becomes noise, right? And, what’s. Really interesting to me is that like all of these pieces of data, they’re all indexed to one another, right?
There are captions pointing to pictures and there are descriptions and hyperlinks pointing to songs and whatnot. and this is what growing AI models eat for breakfast. so maybe we can talk,
about how AI models digest this data, let’s maybe get into the nitty gritty of how they work.
Eryk Salvaggio: Generative AI is based on for the most part, something called a diffusion process. So what we are working with when we work with images and video and sounds for the most part is in the current state of the art, something called a diffusion model. And, this idea is it is diffused, right?
So what does that mean? Essentially what it means is as information that we’ve uploaded, wherever it’s been uploaded, whatever the training data may be. Comes into the model to train the model, information is actually stripped out of it. So if you have an image. It becomes really grainy in steps, and it becomes degraded over the course of several steps, until it is an image of visual static.
A similar thing happens with music. Music is ingested to train the model, and what happens is information is taken out of it, and the same thing happens. It’s noise, and ends up producing a kind of wall of white noise. And video is a very similar thing. With video, it’s blur and noise.
Mack Hagood: I noticed when I was like looking up diffusion models that, you’ll see terms like, like Gaussian noise and Markov chain, but you’ll also need to see words like “destroy”. So, basically is the AI is destroying the image? Is it just gradually turning it from information into noise?
Eryk Salvaggio: It is stripping every possible piece of information out of it in steps. And it does this by following this Gaussian distribution, which means that basically, to be very brief about it, there’s a pattern that the noise follows, and the model learns the pattern of noise being introduced. or, information being removed, same thing.
It follows a particular pattern and because it’s a pattern, it can trace it backward. So you go from an image of pure noise back to the original training image or song. And then what happens is when you generate is it creates a random constellation of pixels and whatever your prompt is, going to try to find a similar path.
Now we’re talking as if it’s one image, right? But it is thousands, hundreds. It’s actually billions of images, in the current state of the art for Stable Diffusion and things like that. So you have billions of images, and so you have billions of paths, and when you type a prompt, you’re basically saying, follow the path that more or less, conforms to the images associated with this keyword or this set of keywords.
So if I say flowers for a prompt, it’s going to this paths of flowers. So it is literally a noise that is at the heart of generative systems. And I think that’s really fascinating.
Mack Hagood: And the example of flowers, you actually have, maybe we can take a look at it. You have a piece where you show this dissolution of an image,
Eryk Salvaggio: so in a demonstration that I do quite often, I start with a picture of flowers. Hypothetically, these are flowers that I’ve uploaded to a social media platform or, a photo hosting website, and I’ve captioned it, “Flowers, somehow, flowers for my sweetheart” right?
Maybe I’m really cheesy on Valentine’s Day. And so this image comes in, and it strips this information out. Some of the first things that go away are the backgrounds, right? It emphasizes like high contrast areas, like the petals in the flowers and the stems of the flowers. And as you go, you realize, okay, I can no longer really tell what the background color was, but I can make out what the petals were.
The really basic shapes stay the longest. And so if you’re thinking about this in reverse, which is the generation of the image, the very first thing that’s generated are these sort of abstract shapes. And that’s what gives the image its structure, but the abstract shapes are just can appear anywhere in this noise.
And there’s another sort of image recognition system that’s saying, yeah, that sort of looks like a flower. and it has to be more and more certain as you go. So the first image that is generated, the first step of this process, doesn’t have to be quite like it’s not going to be a perfect flower.
So maybe the threshold of recognition is like 10 percent chance this thing’s a flower, but every step, the criteria gets a little bit higher. And so what is allowed to pass, is what is recognized by an image recognition system as something that resembles a flower. And so that is how it works.
That’s how it becomes noise. That’s why it becomes noise. And then in the generation process, that’s why you start from noise.
Mack Hagood: So the sort of contours of the argument in the talk, as I recall, is that we’ve gone from this era where a signal or information was this hard, one thing that had to be carefully sifted from the background noise of the universe and the big bang, into this moment where there’s so many signals that they become noise and we struggle to consume it.
We struggle to make meaning of all of it because there’s just so much of it that we don’t, know what to do with it. But. Then we get AI, which knows exactly what to do with it. AI is just like “Yum yum, yum” And just gobbles all of this up. It needs that noise in order to do its own process, which is to reduce images to noise, and then just be able to vomit out exponentially more images.
In theory than what we’ve already placed there. So I guess my question is, what do you want us to make of that? Is this just something that sounds scary? Or is there something in your cultural, social, political critique here that, we really need to be aware of.
Eryk Salvaggio: So my feeling is, you have these machines that are taking in this human generated information. Pieces of cultural expression, text we write, archives are coming in here, and it becomes noise. And there’s something there, right? Is that something that we want this culture to happen to this culture that we’ve produced, right?
That it becomes literally, decimated. And then, what’s an important part of that, is that when we talk about noise, it is a really interesting concept. Because there’s so many definitions of what noise is and how we want to navigate noise and typically, we are in a noisy world, right? We operate in noisy environments.
We have some agency over the decisions we make in terms of what we focus on in that noise and what choices we make in the space of noise that we live in. And one strategy is to think about all the stuff that’s happened before and how that fits the noise and how we can keep following those patterns into the future.
And that is one strategy
Mack Hagood: So like retaining some kind of human meaning? And trying to find, based on our past history, what we value what’s important? Or…
Eryk Salvaggio: Think so if we’re a person and we’re trying to navigate something really noisy and loud and we’re overwhelmed, what’s the first thing we do? Is we look for something that is familiar to us, right? And oftentimes if something scary is happening in the world, we’ll try to fit it to a pattern that we’re familiar with “Oh, we’ll give it a label, right?”
And when we give it a label it helps us navigate and understand that thing, but these labels are this label and prediction response from people is actually what we do when we’re scared, right? And if we are feeling playful and relaxed, Then noise can actually be inspiring and fun.
And we can think about different ways to navigate, like the noise of a party is very different from the noise of a riot, right? If you’re at a party and you’re enjoying yourself, you’re laughing and things are loud, you don’t mind. If you’re in a riot, your thing is look for safety, find the thing.
What do I do? Follow the rules that I have in my head of how to get out of this place, and you focus exclusively on the sort of prior references.
And so what I’m trying to say with this is when we’re building AI, what we are modeling when we’re creating a system that is trying to produce creative resemblance to creativity. Whether it’s in an image or a song, is that actually what we’re doing is training.
We’re constraining all the possibilities of this noise. To the patterns that are learned by the training data. So that if I am trying to create something playful and fun, that breaks the boundaries of say genre or plays with the borders of, what image making can be, right? Something really, truly, experimental and playful and creative in the sense of challenging the past, challenging ideas of, the representation that is present in photography, right?
What have people done before? I want to play with that. You don’t actually get to challenge it. You are constrained to the training data. You are constrained to representations in that training data of what has come before. You don’t really, focus on, when you, get these images back, And there’s these generic defaults, right?
You don’t get something challenging. You get something very comforting. You get something very easy to see. You get something very referenceable because you’re actually navigating it to references, right? You’re asking, you’re using the style of an artist or the genre that exists, right?
There’s limits to what’s possible there.
Mack Hagood: But this is funny because that you’re talking about, getting images that are. average or comforting, normate, so to speak. but I really was excited in the very early days of AI video, because it was so not that. Like the, people I’m forgetting the name of, the one artist who was just being like, there were several people who were doing this, but there was one who was particularly good, who made these just hideously, disturbing, uncanny videos of people morphing. And as the AI struggled
Eryk Salvaggio: Yeah, no, absolutely. So one of the things that I really like about AI is that it’s glitchy. And I think that there’s a lot to explore in those glitches. So even for me, when I’m using an AI system, I’m interested in how does it actually deal with noise? If the entire purpose of this system is to remove noise, what happens if I ask it to generate noise?
And I’ve been able to play with this and what happens is actually it doesn’t reference things in the training data. What it does instead is get confused, right? And I’m personifying it
in a way, right?
Mack Hagood: To clarify, I wanna make sure I understand what you’re saying here. You ask the AI to make
noise?
Eryk Salvaggio: Yeah. So I ask it for visual noise, various types of ways of soliciting images of noise. So you can ask for television static, right? You can ask for things that we know because we know how the system works, that the system is designed to remove. So the system is designed to remove noise. It’s designed to remove static because that’s what it’s starting with, but it’s expecting you to ask for something like a flower or a Rembrandt, right?
It’s not asking, it’s not expecting… the designers were not expecting you to ask for images of digital static and the system cannot accommodate giving
Mack Hagood: you…
What does it show you?
Eryk Salvaggio: What it does is, essentially give you abstraction and it gives you computer generated abstraction. And it’s an artifact of the machine essentially producing something randomly and asking this other verification system in the machine, which is for the techies out there.
It’s clip, right? So clip is trying to say, “10 percent chance that is a flower” But now it’s being asked for a 10 percent chance that it’s noise. But it is noise. It is already noise. So it passes through and then it goes to the next step of noise removal. And so then this originating system has to remove noise from this process.
And then it has to send it over to Clip again. And Clip is like, “Yeah, still noise” right? And so then it goes back and it does another step of removing things towards this abstract concept of what noise is. So it’s actually inflicting a kind of paradox. Into the system, or it’s almost mutually exclusive in a way to say it’s an image of noise, but you have to remove the noise to make the image of noise.
So what you get is never truly a representation of static or digital noise in the way that we might imagine it. It’s always structured based on how the model is trying to remove noise. And the system that’s saying, “Try it again, do it again” Failing. So it’s a kind of feedback loop in the system, but it’s a glitched one.
Mack Hagood: I think I wanted to maybe get you to build this out a little bit in terms of, actual dataset that’s used. You mentioned clip, which I believe is like the machine learning tool that interacts with the dataset. Can you talk about the sort of canonical dataset that all of our AI images are coming from right now? Because I think that’s really fascinating.
Eryk Salvaggio: Yeah. If you’re looking at, the open source tools, which are for the most part, the major one you have right now is Stable Diffusion. A lot of other models exist, but a lot of them are built on top of Stable Diffusion. So essentially Stable Diffusion is if you’re using an open source model, if you’re a company that is starting to work with image generation, you’re taking Stable Diffusion and you’re building on top of it, or you’re, changing it somehow on the backend or you’re fine tuning it.
but Stable Diffusion is like the core. There’s proprietary systems, OpenAI has its own models. We don’t know what’s in there. We don’t know what’s in the training data for those models, Midjourney, we know uses a subset of Stable Diffusions training data. And what this training data is, a data is built on a dataset called LAOIN-5B.
And that is… -5B means 5 billion. It’s 5 billion images that are scraped from the web, all different corners of the web, basically distilled in this process we described. Every one of those images and its captions was reduced to noise. The caption was associated with it as text. And the paths that the noise followed as it degraded that image remembered as an algorithm, essentially like mathematical coordinates.
And transfer it into “the model” as possible paths, which sometimes we call the, vector space.
And so you’re building out these spaces within the model and averaging these things together.
Mack Hagood: A couple of things come to mind here. One is that there’s this kind of a strange irony that AI relies on noise, which is randomness, and yet the way it uses that noise leads to the most average generic. Images possible, right?
Eryk Salvaggio: Because your job is to constrain that noise to its average. What is average in that noise. That’s the goal of the system.
Mack Hagood: And I guess is that why, I might be getting out over my skis here, but is that why they use Gaussian noise? Because Gaussian noise, at least in the sonic domain, is like noise that’s in the bell curve, like the middle. It’s like the average set of noise. It’s, not too high frequency. It’s not too low frequency.
Eryk Salvaggio: So you’re, structurally, already focused on, what I mean when I say the sort of central tendencies. All of this noise is, mashed together, and when you’re generating, you’re finding this sort of average area in the latent space, and then you’re relying on another thing to confirm that indeed is the thing that corresponds to that, right?
An image recognition system, which we know from all kinds of literature around surveillance and stuff, right? It’s pretty biased. and in this case, we’re talking about a bias towards flower shapes, right? But there’s also biases towards people,
Mack Hagood: That was the second thing that I was going to bring up is if we’re talking about averages, we’re talking about stereotypes, right?
And this gets us into, the critiques that a lot of people like, Safia Umoja Noble and the book Algorithms of Oppression, like all these different ways that algorithms rely on datasets that reflect the biases of the past, and then they generate these future biases in these future bias outcomes if they’re not critically engaged with.
Eryk Salvaggio: Absolutely. And so you asked earlier, should we be afraid? I think that this is some of the things we should be thinking about and being critical about. So to take this example, in Dr. Noble’s book, for example, she describes Google search results a couple of years ago, right? And if you did a search of Google for black girls, where did you end up?
The first page of Google search results was pornography, right? Well documented, well researched. And Dr. Noble points this out. And so what does Google do? They changed the algorithm on the back end so that those results are filtered out, right? The thing about it is, that didn’t change the internet.
It changed our access to the internet. And so if you are to look at the training data for these models, and another scholar who’s done great work on this as a Dr. Abiba Barhane, highly recommended. Went in, found all these examples of misogynistic images, racist images, violent images that are in the dataset.
Mack Hagood: This is the dataset that AI that fed on.
Eryk Salvaggio: That was used to train this core image generation model of Stable Diffusion and, there’s, trigger warning, but there was also pornographic contents involving children in that dataset, and that was a huge scandal and as it should be. And so Stanford researchers found this content and they had to take it offline.
The entire idea of having a model like this was that people could go in and audit it, right? Look at what was in there, but there was no mechanism for changing it. And so it was a transparency without any kind of responsibility. but the Stanford researchers, because it was open, they were able to go in and find this content, and now we can’t… actually now we can go in, they’ve adjusted this, they’ve cleaned up that data. So now only in the last couple weeks we are actually able to go and look at what’s in the training data again. But every image that’s in there is contributing to the average that we get out of these systems.
So if you are asking for “black girl” for example, you are getting exactly what Dr. Noble warned about years ago. You are getting pornographic images in the training data. There’s other biases as well that are really fascinating, to look at and think about how they come through and what it means for representation in this space of media in terms of who is defining what a person looks like.
So a classic example is also, and I’m sorry that we’re digging into stereotypes, but I really think it’s an important point. That if you ask for a image of a Mexican person, they will almost undoubtedly be wearing a sombrero, right? This is the idea that it has of images that are labeled Mexican.
And person have sombreros on. Less harmful is a example I did live in Australia. What does a typical Australian look like to an AI system? It looks like a koala. It doesn’t even generate a person. It generates a koala. And because, think about the internet, right? And think about this relationship, this information ecosystem that we’re in and reflecting.
We’re taking these biases. If I’m uploading images that I’ve taken on a trip to Australia, I’m usually not saying here’s an Australian person, right? But if I’m saying Australian, it’s usually Australian kangaroo, Australian wildlife. So these associations are in there. Reduced to noise and then re-generalized .
Through this averaging of all these keywords and all the image data that’s been stripped away of any context whatsoever.
Mack Hagood: The fascinating thing about this to me, or one of many fascinating things is that the data gets generated because what’s happening here is it’s going off of captions of the images, right? And so people are writing and people are going to note a nationality or an ethnicity when it’s the marked category, when it’s the other.
And so you’re actually going to get the perspective of the non- native perspective of Australia. You’re going to get the non- Mexican perspective on Mexico. You’re going to get the non- black version of blackness because if you’re black, it’s not a marked category.
It’s just I took a picture of my sister. It’s not going to be like, I took a picture of my black sister. That is a fascinating and such a glaringly obvious problem that just blows my mind that these tech overlords, never thought about it and/or don’t give a shit.
Eryk Salvaggio: They don’t filter. A Stable Diffusion did not filter the content. That’s obvious, because we had content that is literally illegal in the dataset. So they were not looking at that. They were not curating that dataset. There was no attention paid to what the data was or how it would structure what resulted what emerged from it when it went through this process of, image generation as a model, right?
When it became a model, there was no thinking about what was going to result from that model. But I think the point is, made that fundamentally without this oversight, without this curation of the data that we use, and especially now that most of the conversation around data is how do we get more of it, right?
That’s what the companies are primarily concerned about. When you get more of it, you will also get more bias. And this is actually the subject of that, paper by Dr. Barhane, if you scale, you also scale hate.
That’s the, points that Dr. Barhane makes.
Mack Hagood: So When gave that talk in Poland, you spoke a lot about the difference between a dataset and an archive. And I think this kind of gets to what you’re talking about here. There’s a curatorial responsibility in an archive. There’s an ethics, to how, let’s say, a set of artifacts from that archive are curated in an exhibit
for example. A whole history of people thinking about, people like anthropologists and museum curators and others thinking about the ethics of the archive, especially in relation to our colonial heritage and the ways that things were stolen from, people around the world. None of that ethics of curation seems to be present in these datasets and the people who created these datasets don’t seem to have any concept of this.
Eryk Salvaggio: There’s a myth, I think I would argue, and myth in the sense that it is a shared belief that informs the way that people work and make and do in the AI space. And right now, this myth… And I don’t necessarily mean it’s false. I just mean it is a shared belief among the people building these things, is that the bigger your datasets, the more you scale the information that you train on, the more I hesitate to say this, because it is true, but I think that it to me, it sounds very silly, but almost the more self aware the model becomes.
In a sense that if you gather enough data, you can start asking the data to curate itself, right?
This is this idea…
Mack Hagood: is just the bullshit that they’ve been talking about since Ray Kurzweil with… “We’re just going to get enough computers hooked together that it’s just going to become the singularity and consciousness” it’s like quantity doesn’t scale to intelligence, right? Like these are different things that we’re talking about here.
Eryk Salvaggio: And it is very likely. So scale, we know scale does improve some things, right? But the question is, what does it improve? One thing we have not seen is, that scale reduces the amount of so called “hallucinations”, right? Which is just the machine in, this is an LLMs, right? Large language models. The machines still give bad information. But the argument is that if there’s more data, it’ll stop giving bad information, but we haven’t actually seen that. What we have seen is it gets better at multi-modality, right? I can ask it to turn a white, a physics white paper into a poem, right? It can do that kind of transfer. It can generate more realistic looking text. But ultimately sometimes what it does is generate more convincing texts that’s still factually not referencing the real world, right? It’s not referencing an understanding in any way, because it can’t. Because it doesn’t. But here we’re talking about large language models, which is a whole other can of worms.
But with images to get to this original point, when you’re talking to a museum curator, or you’re talking to an archivist, there is a sense of responsibility. There’s a been a cultivation of ethics, and there’s been reckoning with the failures of those responsibilities and ethics to write, which is really important.
Is that when those things have gone off the rails when, the voice of power is overdetermined what is included in an archive or excluded from an archive, there has been an ability to say, “Shame on you for doing that” With the AI model, who do you point a finger at? It’s almost at this point, you can say, yes, it’s the, people building the dataset.
But they pass it on to the people who are training models on that dataset. And those companies are passing that onto the users who are prompting it irresponsibly. And then the users are saying, “Why are you letting me do this?” and so the blame is, distributed, but I really think that it lays in the foundation of who is gathering this information and who is making the decision to use this information in models that are then being distributed to the general public, right?
That is, there is a responsibility chain there, and we really should be thinking about how do we curate data? And what are the biases of data? But the other problem is you’re not going to solve these issues. You can’t get rid of bias, because there’s always a human making decisions that there are 5 billion images you’re not going to review.
You’re not going to expose to a sort of, auditing process by anthropologists and curators, right? And so there’s some real issues with scale as it applies to this question.
Mack Hagood: In a moment, I want to switch over to something a little more tightly in the wheelhouse of, this podcast about sound, which is how, what you’re talking about applies to music.
But first, maybe we can finish up talking about images by, discussing a piece of yours called “Swim”. And again, maybe for the video podcast, we can, put it up on the screen, but, maybe describe it for our podcast listeners, and non sighted folks tell us what this work is and then, and how you’re trying to engage with the issues that you’re discussing.
Eryk Salvaggio: “Swim” is a nine minute long video piece and there’s three components to it. And so if you can imagine sort of a background, this background is images generated through this glitching process that I’ve described of asking Midjourney in this case, particularly Midjourney. To generate images of noise and actually blending images of noise together, so that what it is trying to do is find patterns in the noise failing and giving me the sort of results, right?
So I’ve taken this failure of the AI system and the result is, in this particular case was a series of, smeary, foggy, almost bubbly looking, digital noise and so this is the background that it’s morphing. It’s, animated.
And on top of that background is an image, I think it’s from the 1920s. it’s an image that comes from the University of Chicago’s video archive of a swimmer. The swimmer is, Ninny Shipley, the film is categorized as erotic entertainment. It is very tame. I don’t want to get the wrong idea of what this video is.
It is a woman swimming underwater, in a bathing suit, right? Full, body bathing suit. And to me, what I’m trying to think about with this process, before I describe what happens in the videos, I wanted to put this image from the archive into dialogue. With an image that was impossible for a AI image generator to generate, right?
So this is the two layers of this piece. and so I took the swimmer and I slowed the swimmer down over the course of nine minutes. And the audio score is also the original sort of jaunty jazz stag party, soundtrack slowed down as well. And it becomes almost, I don’t know. It has this sort of elegiac…
Mack Hagood: The music’s gorgeous. It’s gorgeous.
Eryk Salvaggio: I can’t take much credit for it, but it is a slowed down over. So this 1. 5 minute long piece is slowed down to 9 minutes long, including the audio. And then there’s this 3rd layer, That is turning the process of diffusion, into a visual element of the piece. So over the course of nine minutes, what happens in an instance when you’re training a diffusion model is done over slowly.
And so you’re seeing the disintegration of this image, which is an introduction of noise. So you see the image break apart and you see it break apart into static and the patterns of static emerge, that blends the noise of the background with the foreground of the swimmer. And what I’m trying to do in a way, the way I see it, is I’m trying to use AI and the components of AI that are in the process, almost the sort of machinic artifacts the AI produces, for us to have a way of understanding and visualizing what its interaction is with archives and with history, right? That these things that have an individual meaning and context come up against this impossibility of generating, regenerating something that references it, right? There’s no longer a reference when we break something down.
The flower that is generated has no reference to the flower that I have shared online for my wife on valentine’s day,
right? And that’s a small thing, but there’s bigger things too, there’s history, there’s ways of telling stories that are getting lost in this breakup into noise and separation of image and the labels we assign to image into noise and keywords that gets to, for me, to this distinction between our relationship to something in an archive and our relationship to something that we call a data set.
Which is that a dataset is a thing. We treat it as one thing, but it is actually multiple things that are comprising it, as opposed to an archive where our attention is, what is the history of the objects in this archive? How is that history being told? What are the connections between these pieces? Not just through a lens of which pixels go next to which pixel, right?
Because we don’t care that there are seven images of people in the archive, right? We care about who those people are and what, part of the story belongs to them. With a dataset in this context, we lose that. They just become the shape of people. And I think there’s some grappling that, we can do about that relationship and that transition, especially as we are generating more and more images based on history that kind of erase history at the same time.
There’s a, almost a duty or an obligation of care to what the sources are that formulate that.
Mack Hagood: So let’s talk about sound. Let’s talk about, recent work where you’re thinking about, platforms like UDO and Suno. Do you, want to maybe talk about what those are?
Eryk Salvaggio: Yeah. So it’s, very similar. They’re still diffusion models. They’re still taking representations of sound and they are breaking those down. Now, your audience is probably well familiar with waveforms, but you know for those who aren’t it’s the shape of air, right?
Represented visually. That shapes are the vibration of our eardrums, right? This becomes Just the line, in the training process. And this line becomes the thing to find in a solid white wall of waveform data, which is white noise. And so these models, similar to images, are trained to scrape things away in the direction of, say, a snare drum.
So you get the sort of loud spike at the beginning and the trailing away, right? You carve that out of noise. And so in very short time, what we’ve come to see is the generation of complete audio tracks. So this is, these are the tools that we’re looking at. UDO. Suno, and others, there’s many others, and sure to be many more, that are just oriented around producing a track, a full track, and this is the area that I’m starting to look at now with a lot of interest.
Mack Hagood: Yeah. Maybe we could talk about your experiments with, this kind of diffusion model created music, which is, you have a track called “Sounds Like Music”.
Eryk Salvaggio: Yeah. So “Sounds Like Music” is a reflection of a lot of the ways that I’ve been trying to play with these models. It’s similar to the ways that I was playing with vision or image models, which is to say, let’s try to figure out what the system relies upon and how we steer that system and ways that we might find glitches or find ways of introducing, unexpected user behavior into the system in order to make music that either reflects the sort of process that it uses to generate the audio or emphasizes to the listener what it is that they are exactly listening to. And so I’m trying to think critically about what I’m making and how I’m making it and sounds like music is basically this, concepts of thinking through what is exactly the thing that we are hearing when we are listening to a diffusion based song.
Because, for the first thing, it’s not meant to be expressive of ideas or emotions the ways that a lot of music might be, particularly pop music, right? What it is designed to be , essentially, is plausible. other words, it has to be generated, passed through something that labels it in the context of yes, this is associated with a waveform data that clusters around this idea of, indie or opera or yacht rock.
And this sounds like yacht rock, this sounds like opera, right? The, waveform information resembles the waveform information common to the keywords that have been given to me, which is the genre tag. . The idea is, it has to be plausible. It has to sound like music. And I think that offers a really, pointed way of engaging with what we’re listening to.
Which is, how does it sound like music? And how might it not sound like music? Because the other thing that’s happening, and I think in this song, you can really pick out, pick it up, is that you are taking an image of white noise, and just like we said before, you can reduce that to the shape of a snare drum.
This is generating an entire track. It’s not generating bass, drums, vocals, and then mixing them. It is one giant wall of noise that is being reduced until more or less it sounds like drums, and bass, and vocals. So it’s all one sound that we are listening to that sounds like music. Now what we’re talking about is essentially really compressed, but even with compression, you’re taking different pieces of sound and compressing them into a single wave.
This thing, those forms of, those like lines of music have never been separate. They’ve always been compressed together, and I think this is a really interesting way to listen to this music. You can listen to this song “Sounds Like Music”. And if I would encourage people, if they do to think about how they’re hearing it in the sense of what is it doing to convince you.
That this is music, that this is plausible, right? How is the system figuring out this is passable as music, but also what does it sound like? What artifacts can you hear? What are the decisions that are being made from step to step in this song to produce it according to this constraint of sounding like whatever this genre of music is.
Mack Hagood: This is like getting very much into the weeds and, I don’t want to detract. My question… I love what you’re saying. I’m afraid I’m going to detract from it, but I’m just trying to wrap my mind around… so it’s easy for me to picture the generation of a still image, right?
You’ve got this palette of noise, the constraints of the dimensions of the image you want to generate, and then you whittle away from the image of the flower or whatever, right? Video and audio are a little harder for me to wrap my head around. So it’s do you need to know how long the, song is going to be? And by “you” does the AI need to know how long of a track this is going to be in advance? So basically it’s got three minutes of white noise, and then it’s going to sculpt a three minute trance techno song out of it. You know what I mean?
Eryk Salvaggio: I do. Yeah, you’re, touching a bit on, sound over time, right? The temporality of sound or music in particular, right? And, so there’s, right now, there’s so many different models, it’s hard to give like a one size fits all answer. But what might help is looking at the very beginning of a particular one, which is UDEO and Suno to some degree, right?
So these are the big ones. As far as I’m concerned, that the moments that we’re speaking next week, it might be different, but if you look at the very beginning, what they were capable of doing is generating about a 32 second window and what it would do is essentially it would create this, spectrogram.
And the spectrogram would be a pattern that was associated with 32 seconds of sound because the spectrogram represents sound over time or transitions over time, frequencies and stuff like that over time, you can generate this and then you could transfer that data back by tasting. Okay, this last slice is the last two seconds or whatever, right?
This first slice of the first two seconds and everything in between is distributed evenly amongst that. So you have, an image that is structuring your 32 seconds of sound. Which is what is also really interesting about this current heart of AI is that, it’s an image of sounds that is being transformed to do the sound of sound,
Mack Hagood: Is AI
better at EDM because the, tempos tend to be more standardized. I find that AI seems to be pretty good at creating a drop,
do you find that at all? Certainly when it comes to like vocals, everything gets very uncanny and spooky very quickly, which I enjoy. But some of the techno sounding stuff sounds more plausible to use your word than
Eryk Salvaggio: Yeah.
Mack Hagood: Some more organic music does.
Eryk Salvaggio: I’m speculating, I think a lot of that has to do with the compression, right? A lot of techno is like sounds compressed. They’re using like there’s a noise, there’s an embrace of noise in techno to begin with, to some extent. And the, beat is standard.
I don’t want to, I, don’t want to sound like I’m insulting techno by boiling it down to like standards formulas. But, there are patterns that come, I think, as a result of it being from a machine in the first place. from, the sort of structures of, digital audio workstations.
You have grids that you work with in a, in these things that most people don’t always stick to the grid, but oftentimes, the system is essentially generating the image of a grid. So at the very least, those beats are very simple and it’s identifiable, but I also would push back a little bit on the idea that, I think it’s more plausible sounding, but a lot of the stuff that if you are at the opportunity to adjust what’s called temperature in these audio tracks, the amount of rigidity, is less. Like you reduce the amount of rigidity in the structure, and so you can actually get some pretty wild compositions. But what’s interesting about these compositions is they will still follow, within still I don’t want to say standard deviation, right? But they still cling to the central tendencies.
In the training data. So you can, I call it Deerhoof or Kitsch are the suit to poles. if you’re familiar with the band Deerhoof, they play these like solid rock beats, right? But then suddenly they’ll transform to a techno beat and they’ll be playing jazz over it.
And you can make that kind of thing, but what’s really important distinction is that the machine is not deciding to do that. It is finding sort of the average areas of multiple genres and pasting them together in a way that coheres to the tendencies that have been defined for it in terms of musical structure.
So it’s not creative in the sense that Deerhoof is creative. It
Mack Hagood: I would say maybe it’s even more like 100 gecs. I don’t know
Eryk Salvaggio: Yeah, that’s another good example. Yeah. Yeah.
Mack Hagood: I’m a fan of both so I definitely don’t want to besmirch either band by saying they sound like AI.
Eryk Salvaggio: No, but in a way, what it is getting at is that that’s actually hard for a human to do, right? That’s actually like the sign of a skilled and creative thinker is to think about what your genres are and what those constraints are and how to break them. Whereas what you do with AI is you tell it what the genre is and it tells you here’s roughly, here’s a rough approximation of that genre.
Or you say here’s the genre, but go way out there to these other genres and it’ll find the rough approximation of multiple genres. And then average all of these things together. So there’s no decision making process there, right? It is purely determined by what’s in the training data and how it resembles or how it can be assembled according to prediction.
So it’s, a prediction, right? These patterns of music are studied for patterns because patterns are predictable. And when you ask it to reproduce these patterns, it reproduces predictable patterns. You can assemble those patterns in wild ways, which is where human creativity does come in, I think, but you’re also losing decisions from, moment to moment about what the music is going to do.
So again, it’s about sort of our definitions and our relationships to these things. Like how are we thinking critically about the music? I think as consumers of the music generated by AI, that’s the thing that I want to think about is as a listener, what am I hearing and how do I listen like meaningfully to a piece of AI music that essentially has no meaning.
It’s a reference to the data. It’s a reference to the processes that make it, how do I listen for those things? Which is going to be different from the way you might listen to a piece of music from Deerhoof or 1000 gecs.
Mack Hagood: Yeah. Any meaning is going to have to be attributed to the AI generated content by the listener. And so that really, gets us into the questions of what is music for…
Eryk Salvaggio: And why do we listen?
Mack Hagood: Yeah. Why, do we listen? You use the term hypothetical music. AI is taking noise the noise of human music history, and then putting out some hypothetical offerings for us to do with what we will. In the talk that I saw you give, as I recall, you ended with something of a plea for meaning in this age of noise. And so this idea that we would have to be the ones to bring the meaning to the music, made me think of that. So maybe as we wind down here, can, you talk about how we might restore meaning in an age of noise?
Eryk Salvaggio: My belief is that one of the ways we think about noise is often about how we constrain and control it. And that when we lean on this response to noise, we often encounter problems. Because noise is a part of the world, and it is really the desire for control and definition in response to that noise that introduces, I would say, a lot of problems politically, personally, and socially.
It is not necessarily the noise, but our response to it that creates a lot of these issues, a lot of this, over determination of the possible things that can happen in the world. Because we are uncomfortable by noise. Noise is oftentimes something that we react to out of fear, and so we seek to constrain it.
And AI is a model of that response. It is a desire. It reflects a desire to constrain the paths of possibility to what has come before, to what is in the training data and to predict what might come out of that. If we continued along the lines of the things that have put that day training data together in the first place.
So if you’re generating images, you’re getting stereotypical images. If you’re generating music, you’re getting music that is a reference to patterns found in other music, which was what I mean by the hypothetical. And so what I think is actually the response to noise that we need to embrace is to figure out what are the pieces of the noise, how to hone in on the noise without the elimination of what surrounds it.
What is the context, right? Because right now, what we’re talking about is noise that is made of overwhelming seas of context, a million points of data coming into us at once. And each of those points of data has a context.
And there’s a difference between saying no, to the noise and engaging with the noise. Through a kind of curiosity and engagement. That says this is a piece of noise among many, and I’m aware of the other noise and I’m not going to say it is invalidated or needs to be eliminated or eradicated, right?
But rather that I am engaging with a single point of noise at this particular time and I’m going to give it my attention and I’m going to understand its context. I’m going to think about how this piece that I’m thinking about be of no as noise is a signal and recognize that these are signals, right?
That all of this information coming at us is signal. It’s just overwhelming, but that we can slow down and we can focus on it and we can think about it at a time, one piece at a time without rushing necessarily to cancel all the other noise out. Because we have to learn to live amongst the noise. We need to embrace the fuzziness that we live in around borders and definitions and categories.
And right now, AI is really rigidly tied to these categories and definitions and keywords, right? And it is shaping noise in a way that is aimed at reduction of noise, as opposed to what really introduces the variety and the diversity of an experience of being alive, which is in many ways noise.
Noise can be a source of joy if we don’t respond to it with panic and a desire to control and shape it into something familiar. What if we embrace that noise, try to understand the context that it comes from and think about what we can do from a position of play instead of fear and control. So I say, let’s think about elevating context.
Rather than enforcing control over the noise that comes into our lives.
Mack Hagood: Yeah, that’s lovely. And man, does it sound a lot like the argument I’m making in my book that I’m working on right now, like this book that I’m writing about the history and the future of noise cancellation, white noise machines, algorithmic filter bubbles, all of these different ways that we try to control experiences of noise that we experience.
And paradoxically, it’s this desire to control our surroundings that actually makes us most controllable and actually limits our freedom and limits our ability to be spontaneous, and to deviate from past habits and routines on a personal and a historical level. So I couldn’t agree with you more about what you just said.
And I just want to thank you for being on the show. This has been fascinating.
Eryk Salvaggio: Thanks so much. Lovely to be here. And thanks so much for your work. The noise cancellation things are a real part of thinking about this as well.
Mack Hagood: Yeah. Yeah. we’re, we’ve been like cruising between these different registers of this notorious word noise. But I’m really glad for that sort of multiplicity of noise, because that’s the only way we both wound up at the conference together. So usually I shake my fist at like how, fuzzy it is when people use the word noise.
But in this case, I was like, “Oh…”
Eryk Salvaggio: I’m trying to embrace that fuzziness!
Right? I think it’s important to have a space of fuzziness.
Mack Hagood: Yeah, for sure. That, yeah, that was possibility space of noise.
Eryk Salvaggio: Yeah.
Mack Hagood: All right. Thanks, Eryk. This been a blast.
Eryk Salvaggio: It has. Thanks so much.
Mack Hagood: And that’s it for this episode of Phantom Power. Huge thanks to Eryk for being on the show. You can learn more about all things Eryk Salvaggio at cyberneticforests.com and click on the newsletter tab to get more of Eryk’s brilliance in written form on the regular with his newsletter, see show notes, links, and more for this episode at phantompod.org. Please remember to subscribe to us and rate and review us in your platform of choice and join us at Patreon. We have free and paid memberships where you can get news and exclusive content like the coming backstory on my deal the Penguin. Thanks to my Miami University assistants, Katelyn Phan, Nisso Sascha, and Lauren Kelley for their help with the show.
And I want to give special thanks to Dylan McConnell, AKA tiny little hammers. Dylan created our logo and he also helped me animate it for the digital video age. You can find his, work at tinylittlehammers.com. Thanks also to Josiah Wolf from the band Y. He helped me spice up our intro soundscape with some modular goodness.
So thanks, Josiah. And the one and only Alex Blue, Blue the Fifth did our outro music. Got some feedback on our video turn. Send it to Mack at Mactrasound. com.
This is a work in progress and, oh, and thanks to my dog Pearl for hanging out in the studio with me today. All right. Anyway, peace y’all. Happy new year. See you next month with Liz Pelly.
The post Are AI art and music really just noise? (Eryk Salvaggio) appeared first on Phantom Power.