Cyber Security Weekly Podcast
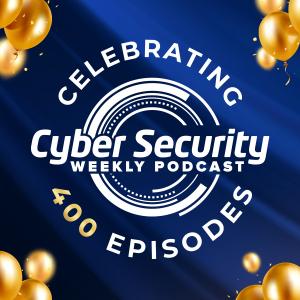
Episode 154 - Taking a data science approach to cybersecurity and threat prevention
Interview with Mauricio Sabena, systems engineer manager, ANZ, Palo Alto Networks, based in North Sydney and discussion on using data science to improve threat prevention.
Using a data science approach to cybersecurity and threat prevention can help organisations detect subtle malicious activity more easily, overcoming the challenge created by cybercriminals’ increasingly-automated approach. Businesses need to understand the potential challenges of using a data science approach as well as the possible benefits, so they can leverage data to outsmart cybercriminals.
Palo Alto Networks has identified four key requirements for a data science approach to cybersecurity:
1. The right amount of quality data. Applying machine learning to data to automate decision-making is an ideal way to combat threats but, if the data isn’t accurate, up to date, or comprehensive enough, the machine won’t learn effectively and the approach won’t work. Likewise, security information and event management (SIEM) platforms aren’t built with the massive computing power that’s required for big data analysis. Running algorithms on big data lakes becomes difficult and costly, and it’s harder for businesses to manage these projects in-house.
Cloud-based solutions can address this challenge because it’s easier to manage resources effectively and elastically in the cloud. Furthermore, customers will depend on security vendors that have huge amounts of high-quality data already, and will let customers run their algorithms on that data. Most security teams only have access to a few weeks of historical data; a vendor-enabled approach will overcome this challenge.
2. Sophisticated algorithms. Data science and machine learning rely on human-made algorithms. These algorithms need to be strong to deliver desirable outcomes. It’s important to put the data in context by looking at all apps, users, and content. This leads to the best quality data. It’s impossible to identify every malicious activity in isolation. Leveraging large amounts of good quality data teaches the machine what’s normal and abnormal. This makes it easier to detect malicious attackers in the network even if they’re exceptionally stealthy.
3. An open mind to false positives. Tuning processes to stop every threat often results in a high number of false positives that must be investigated, leading to unnecessarily-high workloads. Conversely, reducing the number of false positives may result in some attacks getting through. But, with the right data and algorithms, it is possible to lower the number of false positives and get more accurate alerts.
4. Historical records. When it comes to applying data science, historical information is essential. In general, most businesses keep a few weeks’ worth of alert logs, especially if they receive thousands of alerts every day. However, it would be more useful to retain six or seven weeks of data to provide enough of a baseline to determine what activity is normal and what isn’t. Then, when each alert is generated it can be actioned quickly and the security team won’t be overburdened with alerts.
For the full article visit https://australiancybersecuritymagazine.com.au/using-data-science-to-improve-threat-prevention/
Recorded on 22 May, 2019 at Palo Alto Networks, North Sydney.