FourWeekMBA
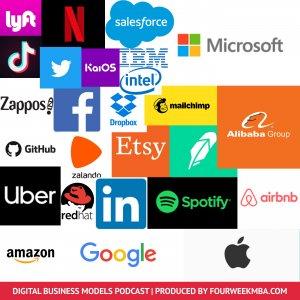
AI Business Models
Nearly a couple of years back - as I saw ChatGPT - just like everyone else who had been in the AI industry for the last decade, it was super clear that it was a turning point.
To be sure, from within the industry, from GPT-2 onward, it was clear that something massive was happening, as for the first time (even if at the time the AI still generated a lot of non-sense), the paradigm was changing, as the output wasn’t any longer stitching together of existing phrases, from a text the AI had somehow found.
But it generated it independently, unsupervised, by “making sense” of the underlying text. That was mind-blowing!
When ChatGPT came out, it was only the confirmation that the underlying model (GPT-3) with a new technique (InstructGPT) could be a game changer.
It's nearly two years after the fact, and we've reached a point where tools like NotebookLM are so impressive that it’s hard to imagine what’s coming next!
Before we get to it and understand its implications, remember you can download the AI Business Models book, if you subscribed to our premium newsletter. As you request access, please provide the email you used to subscribe, and we’ll provide access!
Subscribe to get access to the Book!
Thematic Outline
Fundamental Concepts
A. Technological Underpinnings:
- CPUs vs. GPUs: Differences in processing power, architecture, and applications.
- AI Supercomputers: Role in training large language models, reliance on GPUs.
- Transformer Architecture: Impact on natural language processing, attention mechanisms.
B. Machine Learning Concepts
- Pre-training and Fine-tuning: Building general knowledge and specializing for specific tasks.
- Unsupervised vs. Supervised Learning: Learning from unlabeled data vs. labeled data with instructions.
- Reinforcement Learning: Learning through trial and error, rewards, and penalties.
C. Key Trends in AI
- Content is King: Importance of high-quality data for training effective AI models.
- Multimodality: AI processing and integrating diverse data types like text, images, and audio.
- Emergence: Unexpected capabilities arising from increasingly complex AI models.
AI Business Models and Evolution
A. Historical Context
- The Walled Garden Era: Limited access to information, controlled by portals like AOL.
- The Rise of the Internet: Open access to information, facilitated by web browsers.
- The Reverse Kronos Effect: Startups using technology to disrupt established industries (e.g., Google vs. AOL).
B. Current Landscape
- The AI Ecosystem: Different layers, including infrastructure, models, and applications.
- Business Models in the "Apps' Layer": Ad-based, subscription-based, and consumption-based models.
- Building Competitive Moats: Differentiation strategies and challenges in a rapidly evolving field.
Future of AI & Ethical Considerations
Potential of AI
- Generative AI: Creating new content and pushing creative boundaries.
- InstructGPT: Enhancing AI's ability to follow instructions and generate accurate outputs.
- Decentralized AI Ecosystem: Exploring feasibility, challenges, and benefits.
Ethical Implications
- Bias in AI: Addressing fairness, transparency, and potential discrimination.
- Job Displacement: Analyzing the impact of automation and potential solutions.
- Responsible AI Development: Implementing ethical guidelines, transparency, and accountability.
Summary of the AI Theory Based on Layers, Hardware, Software, and Business Models
The AI Business Models book offers a glimpse into the evolving landscape of Artificial Intelligence (AI), highlighting key layers, technological advancements, and shifting business paradigms.
Layers of the AI Ecosystem:
These can be broadly categorized as:
- Infrastructure Layer: This encompasses the hardware and software foundations, with AI Supercomputers and GPUs playing a pivotal role in providing the computational power needed for training Large Language Models (LLMs).
- Model Layer: This layer focuses on the development and training of AI models like LLMs, utilizing techniques like pre-training on massive datasets and fine-tuning for specific tasks. Generative AI models, capable of creating new content, represent a significant advancement in this layer.
- Applications Layer: This layer comprises AI-powered applications and services that leverage the capabilities of underlying models. The AI Business Models book mentions various business models for companies operating in this layer, including ad-based, subscription-based, and consumption-based models.
New Hardware and Software:
- Hardware: The AI Business Models book emphasizes the critical role of GPUs in accelerating AI workloads. Unlike CPUs designed for sequential processing, GPUs excel at parallel processing, making them ideal for handling the massive datasets and complex computations involved in AI training. AI Supercomputers, equipped with numerous GPUs, provide the necessary computational power to develop and train LLMs.
- Software: The AI Business Models book highlights advancements in AI model architectures, particularly the Transformer Architecture. This architecture, leveraging "attention mechanisms," has revolutionized Natural Language Processing (NLP) tasks, enabling significant improvements in language understanding and generation.
New Business Model Paradigm:
The AI Business Models book touches upon the evolution of AI business models, though they don't provide a comprehensive historical analysis. However, they do highlight the "Reverse Kronos Effect", where startups leverage new technologies and agile practices to disrupt established industries. This effect is exemplified by Google's dominance in the search and advertising market, surpassing previous giants like AOL.
The AI Business Models book also mentions various business models for AI-powered applications, including ad-based, subscription-based, and consumption-based models. This suggests a shift towards more diverse monetization strategies in the AI Applications Layer.
Expected Developments:
The AI Business Models book hints at potential future directions:
- Multimodality: "Multimodality" is a key development in AI, enabling models to process and integrate diverse data types like text, images, audio, and video. This suggests a future where AI applications offer richer and more versatile experiences beyond text-based interactions.
- Emergence: The concept of "emergence" is mentioned in the context of AI. The phenomenon where complex behaviors and capabilities arise unexpectedly from the interaction of simpler components in AI systems. This suggests that future AI models might exhibit capabilities that go beyond their initial design, potentially leading to unforeseen breakthroughs and challenges.
Glossary
Here is a glossary of key terms based on the provided source:
- AI Supercomputer: A computing system specifically designed for AI tasks, using many GPUs and specialized hardware to handle the massive processing demands of training and running large language models.
- Business Engine: The core value proposition and revenue-generating mechanisms of an AI-powered product or service, including pricing models, customer acquisition strategies, and overall business strategy.
- Content is King: This phrase emphasizes the importance of high-quality content in attracting and retaining an audience. For AI, it highlights the critical role of data in training effective models, as data quality and relevance directly influence AI performance.
- CPU (Central Processing Unit): The primary processor in a computer, responsible for executing instructions and managing system operations. It excels at sequential processing, handling a limited number of tasks quickly.
- Distribution Engine: The channels and mechanisms used to deliver AI-powered products or services to end-users, including marketing, partnerships, and platform integrations, facilitating adoption and accessibility.
- Fine-tuning: The process of further training a pre-trained AI model on a smaller, task-specific dataset to refine its capabilities and optimize its performance for a specific application or industry.
- Generative AI: A type of artificial intelligence focused on creating new content (text, images, audio, video) based on patterns learned from existing data.
- GPU (Graphics Processing Unit): An electronic circuit designed for parallel processing. GPUs excel at handling massive datasets and performing complex calculations concurrently, making them suitable for tasks like rendering graphics and training AI models.
- InstructGPT: A large language model developed by OpenAI that uses human feedback to improve its ability to follow instructions and generate more accurate and useful responses.
- Large Language Model (LLM): An AI model trained on a massive dataset of text and code. LLMs understand and generate human-quality text, translate languages, write different kinds of creative content, and answer questions informatively.
- Paradigm Shift: A fundamental change in the underlying assumptions, beliefs, and practices of a specific field or industry. Technological breakthroughs often drive paradigm shifts in AI, leading to new ways of thinking about and leveraging AI.
- Pre-training: The initial training phase of an AI model using a vast, general dataset. This allows the model to learn fundamental patterns, relationships, and representations, providing a knowledge foundation for building more specialized capabilities through fine-tuning.
- Prompt Engineering: The process of designing and refining prompts to elicit the most desirable and accurate responses from an AI model. Effective prompt engineering optimizes AI performance and guides its behavior toward desired outcomes.
- Reinforcement Learning: A type of machine learning where an AI agent learns through trial and error, receiving rewards or penalties for its actions in an environment, allowing it to develop optimal strategies for problem-solving and goal achievement.
- Reverse Kronos Effect: The phenomenon where a startup uses disruptive technology and agile practices to rapidly overtake established industry leaders.
- Transformer Architecture: A neural network architecture that has revolutionized natural language processing (NLP). It uses "attention mechanisms" to process sequential data effectively, enabling breakthroughs in language understanding and generation tasks.
- Unsupervised Learning: A type of machine learning where the AI model trains on unlabeled data, learning patterns and relationships without explicit guidance.