DaVita Medical Insights
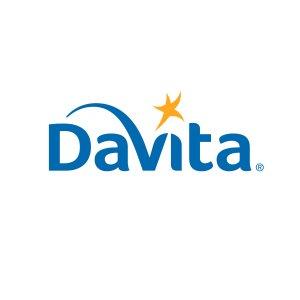
The Importance of Predictive Analytics
Successful use of predictive analytics gives us the ability to minimize unwanted future events and maximize future health without unnecessary expenditure of resources, according to Vice President and Medical Director of DaVita Health Economics and Outcomes Research, Steven Brunelli, MD. Listen to this podcast, in which Ryan Weir interviews Dr. Brunelli on predictive analytics. Dr. Brunelli discusses why predictive analytics are so important; what makes patients with kidney disease well-suited to predictive analytics; how to take a dataset and make it beneficial for patients, physicians and health plans; how an ideal CKD model is defined; how predictive analytics support value-based care arrangements; and finally, how predictive analytics might be used in health care in the future. Listen and read more DaVita Medical Insights here.
Podcast Transcript:Ryan Weir: 00:34 Hello everyone, and welcome to the DaVita Medical Insights Podcast. I'm your host Ryan Weir, and I'm part of DaVita's communications team. Today, we'll dig into predictive analytics with Dr. Steve Brunelli, Vice President for DaVita Clinical Research. Dr. Brunelli, thank you so much for joining us today.
Dr. Steve Brunelli: 00:49 Thanks for having me. It's a pleasure to be here and I'm excited to talk about predictive analytics, which is something that I think is going to be transformative in health care and in kidney care, in particular.
Ryan Weir: 01:00 That's great. Let's dive right in. In health care, everyone is talking about predictive analytics. Could you tell me why predictive analytics are so important and why now?
Dr. Steve Brunelli: 01:11 All right, I'll take that question in two parts. So the first part is: why predictive analytics? The answer is that predictive analytics let us get the right treatment to the right patient at the right time, and that's critically important. As our armamentarium of therapies grow, it's important to be able to target those therapies in efficient ways and in ways that will maximize the benefit to patients. Predictive analytics give us an increasing sophistication in how we deliver those services to patients.
Dr. Steve Brunelli: 01:48 To understand why now, I think it helps to kind of get a historic perspective. So predictive analytics did not spring full form from the head of Zeus. They didn't fall from the sky or come down on stone tablets. They're really an extension and an acceleration in the sophistication of a paradigm that we've used for many years. I liken it to the origins of rock and roll in the 1950s or hip hop in the 1980s. Those didn't derive de novo. They were extensions of prior musical traditions that suddenly accelerated and became exciting and new. That's what we're seeing in predictive analytics.
Dr. Steve Brunelli: 02:29 So what are the permissive conditions that allow for that? The first is we have, by historic standards, an unprecedented amount of data at our fingertips. As electronic health records have increased in their size and their scope, doctors used to scribble notes on index cards and stick them in a drawer in their office, and now everything is on databases in codified fields where people can easily find and index them. Those systems are becoming increasingly interconnected. We have the ability to connect not only various points in health care, doctor's notes, but also labs, observations of the payers like claims. So we have at our disposal now a level of information that didn't exist in prior eras.
Dr. Steve Brunelli: 03:24 At the same time, there's been an explosion of computing power. So the iPhone 5, I'll add, it's in my pocket, is actually a more powerful computer than all the computers that landed the first module on the moon. So when you think about what that allows, and predictive analytics are really an extension of pattern recognition. So doctors have been looking for patterns of symptoms and signs and using that to govern therapies for as long as they've been treating patients. When you take that paradigm and you add that amount of data and computing power at that magnitude, you're able to appreciate patterns in those data and signs in those data that you could never pick up with the human mind. I mean the human mind can only hold about seven pieces of information in active memory and the computer can consider thousands of thousands of those. It can look at combinations of those and the timing of those and identify those patterns in ways that are very powerful and allow us to be much more accurate and precise in the predictions that we make.
Ryan Weir: 04:40 That's great. So what makes patients with kidney disease unique and well-suited to predictive analytics?
Dr. Steve Brunelli: 04:47 So kidney disease, I think, is probably the most fertile field for the development of predictive analytics, and there are a few pieces that go into that. So comparatively, first and foremost, if you want to be able to identify all the patterns, you need data on lots and lots of patients. For better or worse, better in the case of predictive analytics, but worse in the case of overall health of the population, kidney disease is very common. There are tens of millions of people in the U.S. with kidney disease, known and unknown, and that makes it easy to study.
Dr. Steve Brunelli: 05:17 The second piece is that kidney disease and the outcomes that we think about with our predictive analytics, by and large, are relatively objective. So if I want to know if someone has kidney disease, I just need to look at a couple of their labs. Conversely, if I want to know if someone has lupus, there's a whole battery of signs and symptoms and there's various combinations, you assign points. If the patient complains of arthralgia in different points, if they have a rash that's in one part of the face versus the other part of the face. So it's a lot easier when you're training the models in that first step, when you're teaching the models to look for the patterns, when the outcomes that you're interested in are something that are easily definable in the data and are easy to find in the data. So it's easy to find an eGFR lab result. It's easy to find a hospitalization event. It's much harder to find a physician's observation from a physical exam that could be recorded in myriad ways and myriad places in an EHR.
Ryan Weir: 06:28 So it sounds like the data points with patients with kidney disease are a little bit more clear cut.
Dr. Steve Brunelli: 06:32 That's absolutely true. I think the third piece is that, unfortunately, we know that other health events, important health events like hospitalization, like death are common in patients with kidney disease. And again, things being common make it easier to train predictive models to identify the patterns that can then pre-stage those.
Ryan Weir: 06:56 So how do you take that data and make it beneficial for patients, physicians and health insurance plans?
Dr. Steve Brunelli: 07:03 So to build a model, you need several key ingredients. So the first is you need, as we talked about, a large set of data on a large number of patients, and those patients need to be broadly representative of the patients who you intend to use the modeling. You also need the right technologies and the right tech technical expertise to run those technologies. Then the last key piece, and I can't understate this piece enough, is that you need the right clinical expertise to filter down, to oversee all of that, and to make sure that the patterns that you're teaching the algorithm to look for make sense clinically. You don't build in paradoxes, you don't include fluke associations in your predictive models.
Dr. Steve Brunelli: 07:52 So when you take those elements and you put them together, what you do in a large set of data is first you define the population that you want to build the model in. You identify the patients who developed the event that you're trying to predict. So we'll take the case of our hospitalization model. We identify the patients who went on to be hospitalized in some prescribed period of time and, at the same time and by the flip side of the same token, we identify the patients who didn't get hospitalized during that period. So we have our target patients and our controls. Then you step back in time to a period before, the period where you would be making that prediction and you say, "Based on the data, what are all of the things that I could have known about the patient at that moment in time?"
Dr. Steve Brunelli: 08:42 Then through a series of complicated and intricate steps, you teach the computer different ways of looking for patterns between those upstream elements and the event/non-event status of the patient. Typically, so when we built the hospitalization model, we didn't build one model, we built about 65 models. Each one of those went through a series of refinements, an iterative learning by the machine, until it was as good as it can get. Then we had a horse race and we put the 65 models next to each other and we said, "Which of these 65 is the best?" By the best, I mean the most accurate but also the most reproducible.
Dr. Steve Brunelli: 09:25
So when you take a predictive model, it's relatively easy to have it perform well in a development environment, in the population of patients in whom it was developed. The much harder challenge is to take a model that will perform as well in that population as it does into a totally new population. So when we test those models, we test them not only how well do they perform in the development environment, but we export the model to other populations and we look for how well that model can perform externally.
Dr. Steve Brunelli: 10:01 So then you have a model and the question is, well, how does that benefit patients? The first thing, as I mentioned, it's important to be able to identify what patient needs a particular therapy right now. In the hospitalization model, there are a whole suite of services that we can provide to patients: telephonic contact, nurse practitioner interventions, dietary interventions, changes to the dialysis prescription, if we know that that patient is at an increased risk of being hospitalized. So we want to make sure that we're providing treatments that will be effective and help people and we want to minimize the instances where someone gets a therapy that might not do them good in the long-term because the risk-benefit isn't there.
Dr. Steve Brunelli: 10:53 So predictive analytics, by stratifying people into different tiers, how likely are you to have a hospitalization in the next 90 days? Maybe you're high risk and you need an intensive level of services, you need everything we can do. Maybe you're a moderate risk, you need a stepped up level of services. Or maybe you're just in a population average risk and what you need is just solid, every day care, but you don't need those extra services, different changes to your diet, changes to your dialysis prescription. So it helps us streamline that.
Dr. Steve Brunelli: 11:26 From a payer standpoint, be that an insurer, be it a government program, if you're an insurer, you want to know several things. One, you want to know that the resources that you're allocating are being used to maximize benefit in the population it's responsible for. Number two, you don't want to outlay resources that are going to have no benefit downstream. Number three, you want the combination of number one and number two, to prevent future events that will drive up your outlay of costs. So if I can prevent one hospitalization now, I'm going to save as Medicare tens of thousands of dollars two months from now. So I'm very happy to trade off a small investment now to prevent that large expenditure in the future. So from a payer standpoint, these make the delivery of health care and the use of their resources more efficient.
Dr. Steve Brunelli: 12:30 Then I left the providers for last. The providers, be it the doctors, nurse practitioners, physician assistants, nurses, everyone, they live in a world sandwiched between the patients and the health care providers, and the health care insurers, I should say. Predictive analytics provide a way of getting everyone pulling in the same direction. And so it simplifies their job in terms of needing to escalate the level of care in certain patients and needing not to escalate the level of care in other patients.
Ryan Weir: 13:03 So you mentioned that you looked at about 60 plus different models and you went with the best one.
Dr. Steve Brunelli: 13:08 Yeah.
Ryan Weir: 13:08 So what does an ideal CKD model look like?
Dr. Steve Brunelli: 13:13 So an ideal model, so I would say the most important piece for a model, is that it'd be accurate and reproducible. That I can take it in a population of patients, let's say Medicare Advantage patients who, by and large, will be in their late 60s or 70s, and it will work as well on them as it does in an insurer-based health care group of patients who may be in their 40s and their 50s. It'll work as well in a hyper-urban environment as it will in a rural environment and in a suburban environment. So that's the most important piece.
Dr. Steve Brunelli: 13:54 The second most important piece is that a model we built to a very detailed use case. So there are a number of elements that go into that. You need to know what is the outcome that I would like to see from the model? What is the timeline over which I care about that outcome most? I care about it over all times, but there's some timeline that I might care most and I'll give you an example there.
Dr. Steve Brunelli: 14:24 We built a model that looks at the progression of CKD to end stage renal disease. Now, if someone's going to progress, it's important to know that. But as a doctor, as a clinician, if that patient is progressing and they're going to get to ESRD in 10 years, that implies one set of things that I want to do for that patient. I want to take all steps to mitigate the progression of that disease to the best of my ability. But if that patient is going to accelerate over the next 10 months, there's a whole added suite of things that I need to do. That patient needs to be counseled about transplant; they need to be educated about dialysis modalities; they need dietary counseling. There are a whole host of things that I need to do. So specifying the time horizon over which I care about the outcome becomes critically important.
Dr. Steve Brunelli: 15:16 The third piece, and this often gets lost on first blush, but is also critically important, is that when you build a model, you need to understand what the data environment will look like when you go to use the model. So models function like car engines. If you look at the blueprint of a car, there's a series of parts that are connected in a certain way. If you have all the parts, the car runs well, but if you're missing some of the parts, the car won't run. If you're missing a carburetor or, I guess, these days a fuel injector, the car's just not going to run. So it may be the case that if I'm able to feed into a model, build a model that expects lab data, EHR data, and data on social determinants of health, and claims data, I can get to a maximally accurate prediction.
Dr. Steve Brunelli: 16:07 But if in my use case I'm not going to have data on social determinants of health and I'm not going to have claims data available to me in real-time, then that super accurate model can't actually work when I need it to work, when I go out to a use case. So when we build our models, the first thing we do is we work with our partners to understand what will the data environment look like when you go to use this thing. Let's build in everything you'll have access to and nothing that you won't. Then if something changes and there's greater access to more data, we can always go back and build out a later iteration of the model. But we want to make sure that those models are practicably implemented in the use cases so that they can have their intended consequences.
Ryan Weir: 16:57 So with all this modeling and data points and everything, what does success look like?
Dr. Steve Brunelli: 17:03 So success looks like, and I'll give you a general answer then I'll give you a specific example. So what success looks like is the ability to minimize unwanted future events and maximize future health without unnecessary expenditure of resources. We want to be great population health managers, great clinicians and good stewards of health care resources.
Dr. Steve Brunelli: 17:35 So I mentioned that we had built a model that looks at patients on dialysis and predicts their 90-day risk of hospitalization. This model, we knew that technically it was highly accurate from when we built it. We tested it in many separate datasets. It was highly reproducible. We are very pleased with it. But we didn't know if it was successful at that point until we worked with our partners to build out a suite of interventions that link to the prediction the models make.
Dr. Steve Brunelli: 18:10 We let that run, and we let it run for about a year, and then we looked and we said, "In patients who were deemed at high risk, before we started intervening, they, of course had a high risk of hospitalization. We turned on the model and allowed care to be governed by the model. We saw their hospitalization rates deflect downward. When we looked at the medium risk patients who get incremental services beyond standard of care, but not as many, we also saw their hospitalization rates deflect downward. Most importantly, when we looked at the average risk patients, the patients who are the models that were not at high risk, we saw no increase in the rate of hospitalization.
Dr. Steve Brunelli: 19:04 So we were able to prevent this unwanted future event, this hospitalization in the patients who needed that the most and the most vulnerable patients. We were able to do so without adversely affecting the patients who were already in a good place. That's really, in my mind, the key to success here.
Ryan Weir: 19:25 Who else is using this type of modeling today? Can you give some examples of those maybe inside and outside health care?
Dr. Steve Brunelli: 19:23 Yeah. So, first of all, the short answer is everyone is using these models. So within health care, hospital systems use this. Hospital systems are held accountable for readmission rates, patients going back in the hospital within 30 days of having been discharged. So if you're a hospital system and Medicare is holding your feet to the fire about this metric, you want to know if the patients I'm about to discharge, which of those patients are the most likely to bounce back in the next 30 days. So systems will use predictive models to identify those patients who are at higher risk, and then they could potentially offer incremental services, maybe home nursing, telephonic check-ins to try to keep those patients healthy and out of the hospital.
Dr. Steve Brunelli: 20:20 Health insurers use these models. If you've ever been contacted by your health plan about a disease management program or a wellness program, odds are your name came up on a list that was put out by a predictive model to say, "This is someone who might benefit from a smoking cessation class," or "This is someone who might benefit from a gym membership," or whatever it is. So within health care it's very commonplace, but it's even more commonplace outside of health care. The most obvious example is Amazon. When you make a purchase, underneath it says, "You might also be interested in…" All of those things are predictions made by models running in the background. It's not simply a, "We see that you use this product and, therefore, here are adjacent products." It's also they're looking back over your entire purchase history, your browsing history, what items you looked at and didn't buy, the amount of money that you spend, and things like that, and putting all of that into a predictive model to offer you, in this case, a suite of products that are predicted to be of greater interest to you than they would be to the average person.
Ryan Weir: 21:43 Okay. How do predictive analytics support value-based care arrangements?
Dr. Steve Brunelli: 21:47 So first, for the listeners, we'll define the terms. So value-based care is when the risk, the financial risk, for a patient or patients is transferred in whole or in part from the traditional payer to the provider organization. So in the past, if we step back 10 years or 15 years before, value-based care really started catching on. The providers provided services. They were essentially remunerated on a fee basis for the services they provide. All of the financial risk, be it upside or downside, was borne by the payer organizations, particularly in high utilization, high cost sectors of health care like nephrology. Payers obviously want to find a way to hedge against that risk. If they can offload some or all of that risk to provide organizations, they're happy to do so.
Dr. Steve Brunelli: 22:43 By and large, many provider organizations are eager to take on that risk. When you do that, as you know, as a health care provider, your potential downside in the future is remarkable. That if you don't manage patients effectively and efficiently, you'll shut your doors within 12 months or 18 months. That's of concern to the organizations themselves, but also to the patient populations that they serve. I mean, who will dialyze the patients if DaVita doesn't survive or Fresenius doesn't survive. If a regional hospital system shuts down, where do the patients in that community go if they have a heart attack or they're involved in a trauma or they have pancreatitis?
Dr. Steve Brunelli: 23:36 So it's important for these organizations as they take on risk to maximize the likelihood that they can be efficient and effective with their resources. This allows them to hedge against the likelihood of achieving the downside of that risk and the likelihood that they'll achieve the upside of that risk. So it benefits the provider organizations and it also benefits the payers because that's the permissive factor that allows them to shift that risk to the providers. Most importantly, it benefits the patients because in aligning the provider organizations and the payers all to the same true north, which is if you can keep me healthy and keep me out of the hospital and keep me feeling well, everyone wins. That's the magic of value-based care, and predictive analytics are increasingly a very powerful tool that allow us to have everyone pull in that direction.
Ryan Weir: 24:38 So last question. What's the future of predictive analytics in health care and what are you excited about?
Dr. Steve Brunelli: 24:45 So I think the future is almost limitless with predictive analytics. So when we start, when we start in nephrology, so when I look at what are the things that we, at DaVita, have done to date? We've looked at models and analytics that allow us to identify who has CKD but doesn't know it yet. Models that say of the people who have CKD, who's likely to go to ESRD in the near term? Of patients with ESRD, who's likely to go into the hospital? Who's likely to do well on what modality? Those are critically important. Those are mission critical steps along the pathway. That's why we started with those.
Dr. Steve Brunelli: 25:32 But there's no reason why predictive analytics, the efficiency and the effectiveness that they engender, has to be limited to just those critical elements. I think as we all become more familiar and more comfortable with integrating predictive analytics into clinical processes and marrying those predictions to tangible steps that we can take for patients, we can expand that. 70% of the patients dialyze at DaVita have diabetes and a large chunk of those are treated with insulin. Well, even though we're not managing the insulin, insulin dosing is largely arrived at, unfortunately by trial and error. A diabetologist will see a patient, they'll try a certain amount of insulin, they'll see the patient back, they'll review the logs and make the determination either that was too much, too little, or just right, and they'll make an adjustment, and that process will iterate.
Dr. Steve Brunelli: 26:30 Well, wouldn't it be great if we could have predictive analytics that allow us to land on the right dose the first time, or the first or second time, as opposed to the sixth or seventh time and avoid for the patient high and low blood sugars and all the associated symptoms and risks that come with that along the process? So drug dosing I think is a place where we're going to see this. I think oncology is a place where we're going to see, if you have newly diagnosed cancer as a patient, the thing you want most is to know whatever treatment I get first is the treatment that's going to most likely cure my cancer. You can't afford to misstep there. The stakes are very high. So I think we're going to see, those sorts of treatments dictated increasingly by predictive analytics. I think we'll see it filter out throughout decisions, major and minor, throughout the health care system.
Dr. Steve Brunelli: 27:36 But I do want to caution, and I try to always include this when I talk about predictive analytics, that predictive analytics are not a substitute for clinical judgment. They're a tool. You could think about it a tool the same way that a radiology test or a lab test would be. They provide information to a health care provider. That information has to be filtered through that provider's judgment, and they should be seen as a very powerful and potent tool, but not a substitute. That, ultimately, the care of that patient rests with the expertise and the judgment of their health care provider. So as we move forward, to date that has been the case. But I think it's important to always be mindful of that, that there are limitations to what these models can do, at the same time that there's a huge upside to what they promise.
Ryan Weir 28:35 Dr. Brunelli, thank you so much for joining us today. That was some incredible insight on predictive analytics, and I'm sure it's very helpful for our listeners. So thank you again.
Dr. Steve Brunelli: 28:44 Thanks very much. It's been a pleasure.
Ryan Weir: 28:46 Thanks for listening in, and be sure to check out other DaVita Medical Insights episodes for more kidney care-related educational podcasts.