Short And Sweet AI
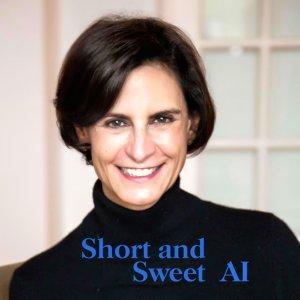
ImageNet
Hi, I’m Dr. Peper and today I’m talking about ImageNet. The story of AI is the story of the pioneers who created it and ImageNet is about a brilliant AI researcher by the name of Fei Fei Li. As always, the links for further reading, videos, and podcasts for this episode are in the show notes.
Fei Fei Lee is considered the rock star of computer vision and articles about her say she started the deep learning revolution and changed everything. As a freshly graduated computer scientist at Princeton, she came up with a revolutionary approach to teaching computers how to recognize images. At the time, scientists were writing computer code, also known as algorithms, to identify cats and then a different algorithm to identify dogs and so on for each object. She thought this was too narrrow. She thought it should be more like how a child learns to recognize images. Children learn to recognize by looking at millions of images. Then she had a brilliant idea: it’s not about the algorithms but the data that you gave the algorithm. So she began to focus on creating datasets.
Datasets
The idea of creating a data base to train computer algorithms to recognize images was considered so ludicrous, laborious and expensive that she couldn’t get funding. In fact an NIH comment rejecting her grant application stated it was shameful Princeton would research the topic. At first she paid undergraduate students $10 an hour to label images but quickly realized at that the slow pace, it would take nine years to create the data set and so the project stalled and languished until a chance conversation with someone who suggested she look at Amazon’s Mechanical Turks. The Mechanical Turks is a system of workers worldwide being paid very small amounts to do piecemeal work. This was a breakthrough for hiring a cheap, fast labor force to label the images. Even so it took another two and a half years to amass the initial 3.2 million images called ImageNet.
Li and her team then offered their dataset to an image recognition contest. In the competition, AI researchers would use their newly developed algorithms to see how accurately they could identify the images in ImageNet. In the beginning the best algorithms in the contest could identify the images with only 75% accuracy. Then in 2012 something very big happened. Researchers won the contest using a type of deep learning algorithm called a convolutional neural network with amazing accuracy. And each year after that the neural networks improved until the accuracy was 98%. In effect computers could see better than humans.
Data = Fuel
The 2012 event triggered a wave of excitement. There was a huge acceleration in using deep learning and convolutional neural networks which launched a revolution. ImageNet changed the field as people realized the thankless task of making a dataset was at the core of AI research. It wasn’t just about the algorithm or neural networks.
Today ImageNet has 15 million labelled images and large companies such as Google and Facebook have created their own datasets of voice clips, text snippets, even video datasets of people performing tasks. Datasets are the fuel for the different deep learning neural networks which have ushered in new technologies such as advanced smart phone cameras and self-driving cars.
And it all started with Fei Fei Li and her quest to teach machines to see.
However, as with all technology, there are unforeseen consequences, the unknowable unknowns. And in my next talk we’ll see how ImageNet has become the poster child of what bias in AI l...