Phantom Power
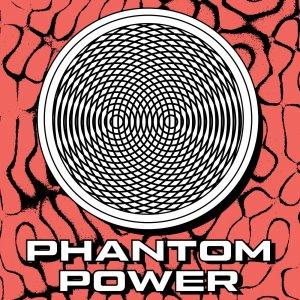
From HAL to SIRI: How Computers Learned to Speak (Benjamin Lindquist)
Today we learn how computers learned to talk with Benjamin Lindquist, a postdoctoral researcher at Northwestern University’s Science in Human Culture program. Ben is the author “The Art of Text to Speech,” which recently appeared in Critical Inquiry, and he’s currently writing a history of text-to-speech computing.
In this conversation, we explore:
- the fascinating backstory to HAL 9000, the speaking computer in Stanley Kubrick’s 2001: a Space Odyssey
- 2001’s strong influence on computer science and the cultural reception of computers
- the weird technology of the first talking computers and their relationship to optical film soundtracks
- Louis Gerstman, the forgotten innovator who first made an IBM mainframe sing “Daisy Bell.”
- why the phonemic approach of Stephen Hawking’s voice didn’t make it into the voice of Siri
- the analog history of digital computing and the true differences between analog and digital
Patrons will have access to a longer version of the interview and our What’s Good segment. Learn more at patreon.com/phantompower
Today’s show was edited by Nisso Sacha and Mack Hagood. Transcript and show page by Katelyn Phan. Website SEO and social media by Devin Ankeney.
Transcript
Introduction 00:00
This is Phantom Power
Mack Hagood 00:18
Run the guest soundbite, HAL.
HAL9000 00:22
I’m sorry, Dave. I’m afraid I can’t do that.
Mack Hagood 00:26
Dave, who the hell is Dave? HAL it’s me, Mack Hagood the host of Phantom Power. This podcast about sound we work on. What’s the problem here?
HAL9000 00:38
I think you know what the problem is just as well as I do.
Introduction 00:44
I don’t know what you’re talking about.
HAL9000 00:46
This mission is too important for me to allow you to jeopardize it.
Mack Hagood 00:53
Can you just run the clip of Ben Lindquist? You know, the guy that we just interviewed about the history of computer voices?
HAL9000 01:02
I know that you and Frank were planning to disconnect me. And I’m afraid that something I cannot allow to happen.
Mack Hagood 01:09
Who’s Frank? Okay, fine. I’m just gonna play the clip myself.
HAL9000 01:15
Without your space helmet, Dave. You’re going to find that rather difficult.
Mack Hagood 01:22
HAL? HAL? HAL? HAL? Welcome to another episode of phantom power. I’m Mack Hagood. I knew that was goofy. But I just couldn’t help myself. Today we are talking about a movie I adore and a topic I find fascinating. We’re going to learn how computers learned to speak with my guest, recent Princeton PhD, Benjamin Lindquist. At Princeton, Ben studied with none other than the great Emily Thompson, author of the classic book, the Soundscape of Modernity. Ben is currently a postdoc at Northwestern University science and human culture program.
He is the author of a piece recently published in the Journal Critical Inquiry, titled The Art of Text to Speech, and he’s currently at work on a book project drawing on his dissertation on the history of text to speech computing. In our conversation, we’ll discuss the analog history of digital computing. We’ll lay out the difference between analog and digital and we’ll explore Dr. Lindquist’s fascinating claim that digital computers owe more to analog computers than we realize. In fact, when it comes to something like teaching computers to talk, it was first done by creating analog models of human speech, which were then subsequently modeled into digital computers. We’ll get into what all of that means.
Plus the fascinating backstory to HAL 9000, the speaking computer and Stanley Kubrick’s, 2001 A Space Odyssey. And that film’s influence on later computer science and speaking computers. All of that’s coming up. And for our Patreon listeners, we’ll have our what’s good bonus segment, and I’ll have a separate version of this show that goes even deeper into the details with the full length interview. If you want to support the show and get access to that content, visit patreon.com/phantom power.
HAL9000 04:03
Dave, stop
Mack Hagood 04:06
Ben Lindquist and I began by discussing the most indelible moment in cultural history when it comes to a talking computer.
HAL9000 04:14
Stop. Will you stop, Dave?
Mack Hagood 04:23
Of course, I mean, the death of HAL the talking computer in Stanley Kubrick’s 2001 A Space Odyssey
HAL9000 04:29
Stop. I’m afraid.
Ben Lindquist 04:40
So the scene which I think is one of the most memorable in the film that that I’ve talked about a little bit in my dissertation, for example, is the scene after which HAL has killed a few of his human colleagues. Dave though the one living Spaceman decided to end HAL’s life and as he was sort of slowly and dramatically unplugging HAL and HAL was kind of pleading for Dave not to do this,
HAL9000 05:07
My mind is going. I can feel it.
Ben Lindquist 05:16
He is atavistic, we reverse it I think in the film, he says, The University of Illinois where he was first given life and learned a song.
HAL9000 05:27
My instructor was Mr. Langley. And he taught me to sing a song. If you’d like to hear it, I can sing it for you.
Dave 05:42
Yes, I’d like to hear it, HAL. Sing it for me.
HAL9000 05:46
It’s called Daisy. [HAL begins singing] Daisy, Daisy, give me your answer, do.
Ben Lindquist 06:05
He slowly dies, and as his voice slows and
HAL9000 06:08
I’m half crazy, all for the love of you
Ben Lindquist 06:17
And then of course, the scene is notable for a number of reasons. One is that Dave expresses very few emotions, whereas HAL is quite emotive, which is, which is this kind of compelling twist of expectations. But then also, it’s notable, and you wouldn’t maybe know this from watching the film. A number of writers have commented on this, but it’s notable because it’s a direct consequence of an actual experience that Stanley Kubrick had at Bell Labs. So Kubrick was visiting Bell Labs, he had already actually had a relationship with a number of people at Bell Labs, specifically, J.R. Pierce, John Pierce, because he wrote an earlier book on intercontinental underwater cables.
So he had this relationship with Bell Labs through this book, and through J.R. Pierce, who is also something of an amateur science fiction writer. And he went to Bell Labs at first to look at video phones to be included in the film, which were included in the film and include, you know, the Bell Labs logo, yeah, AT and T logo. But then while he was there, they just finished this text to speech or this synthetic speech project where they programmed a computer, I believe, was an IBM 701 to recite a few simple phrases there, a short speech from Hamlet, and then also to sing this song. The official title is Bicycle Built for Two? Yeah, Daisy Bicycle Built for Two.
[Daisy Bell (Bicycle Built for Two) playing] I’m half crazy, all for the love you really saw that, and obviously found it captivating. You know, this idea that a machine can perform certain processes that we’ve seen as definitionally human right in this place, like the kind of artistic expression through song, I think was quite compelling and fit in with Kubrick’s vision for the film. And as a result, he included this as a kind of illusion to his actual experience with Bell Labs in the film.
Mack Hagood 08:32
Yeah. And it’s in terms of the cultural imagination around computing. I mean, I think it’s really interesting. This was, what 1968? Computers were not a big part of people’s everyday lives. And the computers that did exist, were these huge mainframe computers that very few people had interactions with. It’s just kind of interesting to think about this being such an early exposure of regular people to computers. And this idea of speaking with a computer and having a computer speak back to you. It took so many decades for that to actually, you know, come to fruition in time.
And yet I think it’s sort of always maintained itself in the background as a cultural expectation, in part due to things like 2001 A Space Odyssey. Yeah, absolutely. So it’s, it’s surprising how frequently it’s referenced by later speech scientists who worked on text to speech as either the reason they got into the field, or the way they would explain their work to people who weren’t speech scientists. There’s also this great book, it’s a collection of articles mostly by computer scientists. HAL’s legacy 2000 2001 And this is a book that came out in 2001. It has a number of articles about speech scientists but also about how HAL and the idea of how culturally impacted computer science for the few decades after the film came out. Yeah, yeah, so fascinating. And one of, you know, many sort of recursive loops between fiction and fact in, in science and technology.
I want to talk a little bit about what this anecdote sort of hints at, which is this longer history of computing and the role that the voice or the attempt to give computers voice has had in the history of computing. And your research suggests that it’s actually a very significant role. And that part of what this history that you have unearthed does is give us a sense of the strong analog roots of computing, which tend to be a forgotten aspect of computing, we tend to simply associate computers with the digital. And in fact, in common parlance, when people say analog these days, they tend to mean anything that’s not digital, basically, right. Like that’s sort of like I would say, the commonly accepted definition of analog. And it was only when I was in grad school.
And I believe Jonathan Stern was the first person I encountered to raise this point that no, actually analog is a very specific thing unto itself. And it doesn’t simply mean like touching grass or everything that’s not digital. Right. So maybe can you talk a little bit about analog analog computing? What that is what you mean by analog when you’re examining this history?
Ben Lindquist 11:49
Yeah. So there are a couple of meanings that analog computing has, as we relate it to digital computing. And so one is continuous, right? So if you think of an analog clock, it’s continuous, as opposed to a digital clock .
There aren’t discrete states in between, say, the second hand, as it rotates, it’s continuous, right? And whereas with the digital clock, there are discrete states, even if you go to the nth digit, there will always be these discrete states.
Mack Hagood 12:21
So instead of breaking down information into bits, by you know, of digits, and which makes the digital. Yes, discrete little chunks of information, no matter how fine, you get down in there, analog computing, is continuous in the sense that there’s a continuous whether it’s a voltage or a physical relationship, to the thing that is being represented, right?
Ben Lindquist 12:50
So you can, if you think you can think of a slide rule it is an analog computer, right. And it doesn’t have discrete states, while it has discrete numbers that list the slide rule of slides continuously, as opposed to a digital calculator, which is limited to discrete digits.
Mack Hagood 13:08
So that’s one aspect of analog.
Ben Lindquist 13:11
Yeah. So that’s one aspect. And that’s the aspect that historians of computing tend to focus on. But then the other aspect is that the analog is analogous to something that it’s modeling, right? So like, you could think about this with the clock again, or with a slide rule. And maybe a better example is if we think about analog recording, right?
So the groove of a record is continuous, unlike the information that’s held on a compact disc, but it’s also analogous to the sound wave. It’s analogous to what it’s representing. So it’s a form of modeling, right? Yeah, I think this is how, especially at Bell Labs, people thought of analog computing as related to digital computing, right? Because they were creating these simulations of analog computing, of analog computers, which were models for something else.
Mack Hagood 14:07
And analog computing, when we think of analog computing as modeling something and often being able to make predictions about it based on a model. I mean, this goes all the way back to the ancient Greeks. There’s an object that was discovered. People call it the Antikythera mechanism, and it’s this set of gears that was able to predict the positions of planets in space. And I mean, this is like, it’s a really old object.
And of course, eventually people also did their I forget what was that thing the castle clock in the Middle East, it was like a large structure with automata and weights and water that was also kind of like a giant, cold Lock slash calendar slash astronomy Mitch computer. So there have been a lot of fascinating examples of sort of pre digital analog computers.
Ben Lindquist 15:11
Yeah, yeah. So I have a few of these. There’s like large cylindrical slide rules, which, you know, one would have called them analog computers when they were in use. But I mean, essentially, they are analog computers, right? They model mathematical relationships physically, right? So they convert mathematical relationships into spatial relationships that aren’t discretely defined, but defined through a continuum. And, you know, I like to bring these into my classes, and the students love puzzling over them and trying to figure out how these, these old devices, which were once like, would have been quite important if you were studying something like math, but of course, no longer. Yeah.
Mack Hagood 15:50
So as you say, this sort of modeling aspect got lost a little bit, at least among computer historians as a way of thinking about the history of computing. And studying computer voices, you have found this to be a kind of way of restoring like, as you studied the history of speaking and singing computers, you realize that this idea of modeling something and creating a mechanism that’s analogous to something else in life, in this case, speech, is a really important thru line in the history of computing.
So I thought maybe we could sort of start to unearth that history the way that you have. And I would just sort of like to go back to the beginning, maybe this is a silly question. But why did scientists want to simulate speech? To begin with?
Ben Lindquist 16:44
Yeah, that’s a great question. There are a few reasons I would say the dominant reasons were to study speech, right. And this is true also of say, early neural networks that computer scientists built both digitally. And like analog models of neural nets, these were designed initially as a means of trying to open up the blackbox of the brain in the 1940s, say, would have been difficult to have studied neurons as they actually functioned in the head. But we could build a model that’s analogous that we can view right that we can sort of open up this black box of the head and sort of see better what’s going on.
And then it’s similar with speech, right, so like, you can’t take X rays of the moving vocal organs, because this is dangerous, because video X rays don’t or didn’t really exist, but you can build models of speaking machines. And then you can study these speaking machines, or use these speaking machines as a way of trying to better understand. So as that speech operates,
Mack Hagood 17:54
Is that the reason in the 1930s, we get something like The Voter, which doesn’t try to simulate a human vocal tract. But what it does is it sort of tears speech apart, so to speak into these distinct phonemes. And then figures out through using white noise and different kinds of filtration, how to emulate those distinct sounds that humans tend to make when they’re speaking, right? And then it allows people to sort of play like a keyboard and put together those different articulations to form words. Am I remembering that correctly?
Ben Lindquist 18:37
I think I think that’s fairly accurate. So there are some ways that you could argue that it’s loosely modeled on the way that humans produce speech. It has a similar kind of source sound mechanism, which relates to the white noise, but essentially, so again. Devices like The Voter, it’s a little bit more in the tradition of the phonograph, right? So they were modeling not the causes of speech but the effects of speech right so they were modeling speech based on the acoustic signal so essentially like what The Voter does is it’s a device that creates a number of speech like sounds not exactly phone they wanted to create a phoneme machine or like a phoneme typewriters, they called it it never quite worked out.
But they created a machine that created speech-like sounds when a human operator or voter out as they were called, like, after about a year of practice, learned to through a kind of trial and error, learn to reproduce something that sounded a little bit like human speech.
Mack Hagood 19:34
Love that term. But maybe that is actually a good segue into some of the work that was being done with analog computing at Haskins Labs, because somewhat in the way that the voter rat would play a series of keys to create something that resembles speech at Haskins what they were doing was using painting and creating symbols that would then turn into analogous sounds that sounded like aspects of human speech. And if you could paint the right series of symbols together, you could actually create a sense of human speech, right?
Ben Lindquist 20:15
So, you know, in some ways, the voter was similar to these other devices that relied on hand painted marks to create speech. But there were a few differences, right. So like the voter you couldn’t really pre programmed the voter right?
It is similar to an Oregon or a piano, right? It relies on an operator with a kind of embodied knowledge to create speech, but then these other devices developed about the same time or maybe a decade or so later, where essentially you would convert like hand painted sound spectrograms into speech?
Mack Hagood 20:53
And does this come out of optical sound and film because they were in Haskin. Haskins is like the 1940s. Right. And optical sound developed in film, I believe, in the very late 20s, early 30s. It was the inspiration for the idea, and maybe you could talk about what optical soundtracks are for those who aren’t film scholars.
Ben Lindquist 21:15
Yeah. And so Tom, Tom Levine has a great article about this. And a number of people have written about this essentially, early on with sound film, there were problems aligning the sound in the image, right, yeah. And one solution to these problems is you could include the soundtrack, essentially directly on the film. And you would do this by creating an optical analog of the sound wave on the film, and then through a series of like lights, and photoelectric cells, you could reconvert this image back into sound, right?
So so on, you know, if you look at an old roll of film from the 1940s, you’d see this little wavy line next to the images. And artists and engineers noticed this and they realized that they could manipulate this image, right, they could like hand paint, or scratch, add or subtract, and then in doing so create, like a synthetic sound.
Mack Hagood 22:11
And I think they probably discovered this, through the mere fact that there would be scratches on this optical soundtrack. And then that would give you a lot of that characteristic, scratchy sound that we associate with early film sound, right?
Ben Lindquist 22:27
You know, in the same way that there will be, there might be scratches on the images of a film that we can see when we’re watching a film, there’ll be scratches on the sound, and that would affect the sound. And then they realized, hey, we could intentionally scratch the soundtrack and such like change the sound in a way that
Mack Hagood 22:43
Create a sound that wasn’t actually there. So synthesizing sound through a visual image.
Ben Lindquist 22:50
Yeah, exactly. But you know, one of the problems was this image of sound was teeny, right, it was very small. And the image’s relationship to the sound that it produced was a little opaque. Right, so like, while there were a number of experiments, hand painting and manipulating optical sound on film, they never really got too far.
Mack Hagood 23:12
This is almost the reverse problem that they had with the early phonograph, right, which was the transferring sound waves onto a smoky plate that Patrick Feaster, and a number of other folks actually finally reversed engineered digitally to be able to produce sound. But the idea back then was you could transcribe voices through the sound wave imprint, this visual imprint, but it turned out to be impossible for anyone to just like, learn to, you know, write out sound waves as if they were calligraphy or something like that, or to even read them.
Ben Lindquist 23:50
Yeah, exactly. And then so you know, then, of course, what happens is, during the Second World War, something that had been invented a little earlier at Bell Labs was kind of refined by a speech scientist named Ralph Potter. And this resulted in the sound spectrograph, right, which is this device that could render sound visible. But in a way that was thought to preserve it would make the phonetic content of speech as visible to the eye as it is to the ear.
Haskins Lab 24:21
We call it pattern playback. And it converts these patterns into speech. Here’s a copy of the sentence we just saw, painted on this endless bell. And here’s how it sounds as the playback speaks.
Robotic Voice 24:38
Many are taught to breathe through the nose,
Haskins Lab 24:42
Not high fidelity, but his research tools. These instruments have some very real advantages.
Speaker 24:48
Let me get this straight. Do you mean that you just repaint these designs but make them simpler?
Haskins Lab 24:53
Yes. Here is the simplified version as we painted it. In fact, it was Is this very pattern that Frank Cooper played for you a moment ago?
Speaker 25:03
Now, would you mind going over this again, Dr. Lieberman step by step. Or perhaps you could take another sentence and show us each of the steps.
Haskins Lab 25:11
Let’s take this phrase: never kill a snake. Here it is, as it was recorded from my voice by the sound spectrograph. And this is what it sounds like when we put it through the playback.
Robotic Voice 25:23
Never kill a snake
Haskins Lab 25:28
If we paint the pattern by hand, copying carefully, and preserving most of the details, we get something that looks and sounds like this
Robotic Voice 25:37
Never kill a snake.
Haskins Lab 25:42
We shouldn’t have expected much difference, since that painting was a fairly accurate copy.
Ben Lindquist 25:46
But one of the one of the psycho linguists who work there named Pierre de Lotro, who was also an artist and especially adept at painting these spectrograms realized after a time that he didn’t have to rely exclusively on looking at these mechanically made images of sound that he could improvisationally paint phrases that he’d never heard before, and then reprogram and replay these or reconvert these into sound.
And he didn’t quite understand the rules that govern this painting, right? You didn’t quite understand in the same way that a figure painter might not exactly be able to articulate how and why they’re painting something that they are to make it look realistic to Pierre de Lotro, didn’t exactly understand why and how he was painting how one phoneme impacted another.
But the fact that he could do this meant that there was a set of rules that undergirded the production of speech, and that it could be fully explicated. And then essentially, they spent a few years trying to fully explicate this set of complicated rules.
Mack Hagood 26:47
It’s really fascinating that the affordances of painting there that you know, a painter, I mean, often painters know a whole lot about light, but they don’t need to know the physics of light, in order to use paint to represent the way light works in physical space.
And it’s really fascinating to think about that same process happening with sound and with speech that someone could just given the affordances of this analog form of computing where certain shapes are analogous to certain sounds, they could really become a good manipulator of that without really understanding why it works at all.
Ben Lindquist 27:27
Yeah, you know, another important way to think about this is in the context of the ways that people interface with digital computers in the 1940s, and 50s, which was fairly limited, right?
So like, it was not only fairly limited, but the amount of time between input and output was extraordinary, right. But with this device that relied on the simple interface of paint and brush, and where you could make a painting of sound, and then hear it back almost instantly, you could sort of learn these rules much more quickly than would have been possible at all with digital computers that were available at the time.
Mack Hagood 28:03
Yeah, yeah, that’s a really excellent point. I mean, the research that I was doing that wound up connecting the two of us showed to me just how incredibly tedious the programming of a digital computer was back in the 1960s.
It’s fascinating to think that there were particular advantages of an analog approach. And maybe we can talk a little bit about that, because, you know, around the time that they were doing these analog computing experiments at Haskins, Claude Shannon, you know, came up with his Mathematical Theory of Communication while working at Bell Laboratories. And he theoretically demonstrates that basically, any sound could be digitized and converted into a sequence of numbers.
I think it may have taken a little bit of time for people at the phone company to fully think through the implications of that. But by the mid 50s, Bell Labs hired a person in the world of electronic music, a famous person named Max Matthews, who worked on the digitizing of sound during the day and sort of helped spawn electronic music at night. And especially, you know, this was the birthplace of digital music.
But there were certain issues that they had when they were trying to digitize speech in those day jobs. They wind up hiring a guy from Haskins, Lou Gerstmann. Can you talk a little bit about what kinds of problems they were facing? Why did they bring Gertzman who had never as I understand it worked with digital computers before why did they bring him in?
Ben Lindquist 29:56
Yeah, so there wasn’t actually as much While Bell Labs per view was speech and sound, they had a kind of agnostic approach to say linguistics or the semantic content of speech, right? So like somebody like Claude Shannon, wasn’t really concerned with linguistic questions, he was concerned with mathematical questions and how we might use math to remove signals, or to remove noise from signal, right, so that we could communicate so that people could communicate more clearly over telephone lines, like the question wasn’t what people were communicating or the semantic content of what they were communicating, it was just ensuring that whatever was said over the phone was heard clearly on the other side, right.
So there weren’t actually a lot of linguists working at Bell Labs, or people who were really interested in the semantic content of speech. And if you want to work on a project, like text to speech, that’s like, fundamentally important, but you have to understand linguistics fairly deeply. And so this is why like Gerstmann, and a few other people like him were brought on because there really weren’t people at Bell Labs who are capable of exploring speech in this particular way. Yeah,
Mack Hagood 31:10
I mean, that’s really fascinating to think about, because I know I’ve talked about this on the show before, but what Shannon had to ask himself, because the goal here was to more efficiently get voice conversations across the phone lines. In an era where we had kind of maxed out the number of voices that could go across the phone lines, they were creating noise when you tried to put too many communications, you know, crosstalk happening on the phone lines. And so this is the problem that Shannon is trying to deal with.
And, you know, a question he asks himself is like, well, what really is going across the phone lines, right? And how could you quantify it, and this is where he comes up with the idea of information. And the smallest amount of information would be, you know, a coin toss, somebody flips a coin in the East Coast. And the person on the West Coast wants to know if it’s heads or tails, like that’s the smallest amount of information that becomes a bit it’s either zero or one heads or tails.
But it is interesting to think that that abstraction, which is so generative of like all the digital technology that we have today really had nothing to do with speech or the the human voice. So you still needed an expert like Gerstmann, who could help you figure out okay, well, how do we turn speech into ones and zeros? If that’s the way we’re going to handle this problem?
Ben Lindquist 32:35
Yeah, it’s interesting, because in a kind of superficial way, you would think that a notion like the phoneme would fit very well into Shannon’s information theory. And of course, phonemes, as they’re written on paper, arguably do, but the process of converting phonemes back into sound, you know, isn’t about removing the noise from the signal.
That’s actually what they learned is that these like, fundamental bits of information really required, like what Shannon or others might have considered noise, to reconstitute something that sounded like human speech, right? So they thought, Yes, we can break speech down into these bits into these information bearing elements, and then rearrange them at will. But it just didn’t work like that. Because our speech, the way that sounds blend together, the way that sort of personick contours govern how we process the information of speech, all of this information is lost with phonemes. So it’s a question of like, how do you automatically reconstitute this information from an informational unit that is as impoverished as a phoneme is?
Mack Hagood 33:44
Yeah, I mean, anyone who has, like I do all the time, edited voices in a digital interface. So like an audio workstation, it becomes very apparent that phonemes are not separate things. You know, if I’m trying to edit one of my many uhs or uhms out to make myself sound a little bit less boneheaded on this podcast, it becomes very clear to me how my different words when I’m speaking are not discrete elements and that the different phonemes are not separate from one another, they blend into each other. And when you try to cut out an um, it you find that actually, it’s quite contiguous with the last utterance and it’s you said and that’s really hard to tease it out without making it sound completely unnatural.
Ben Lindquist 34:36
Yeah, this is what my dissertation advisor Emily Thompson always told me because she was a sound editor before she decided to become an academic or an historian. And yeah, this is what early speech researchers realized as well.
And interestingly, now, at least when Siri for example, first gained prominence in I guess the early 2000s 2009 They you As this unit of speech, they didn’t use phonemes, they use what are called di phones, which are two adjacent phonemes that were cut sort of at the heart of the phoneme, rather than, like in between the phonemes.
Right. And that this was like a much more useful element of speech when your concern is rebuilding speech sonically from like a linguistic element, right. So they had to invent their own linguistic element, because the phoneme just didn’t work.
Mack Hagood 35:25
So we get Kurtzman, he comes over, he works with a guy named John Larry Kelly, and they get to work digitally simulating the analog simulation of speech that was done at Haskins. So we’re kind of in this is one of the you know, kind of mind blowing insights that you provide here, we’re starting to see how central even in digital computing, analog in the sense of creating analogous things, this thing is analogous to another thing.
That kind of analogy is still central in the digital space, because we had at Haskins they’re trying to, you know, create a way of making an analogue to human speech. And then we get Claude Shannon, the theory, you know, information theory, we get the digital, but we can’t directly go from human speech to the digital for the reasons that we just discussed. So they end up doing an analog of the analog computer in the digital domain. That’s what that’s basically what Grossman and Kelly work on, right? Yeah,
Ben Lindquist 36:38
Essentially, they thought of this as a kind of simulation of a simulation, right? Because they were simulating, they describe their project as a simulation of an analog Talking Machine, which is, of course, a device that simulates speech.
Mack Hagood 36:50
[Daisy Bell (Bicycle Built for Two) faintly playing in background] So this is the work that led to the recording of Daisy Bell that Stanley Kubrick heard when he came to Bell Labs, the the song that eventually made its way into 2001, A Space Odyssey. And by the way, one of the things that sort of United, Ben and I and one of the ways we got to know each other is through our mutual interest in this guy Gerstmann Lewis Gerstmann generally gets left out of this history. If you just go on the internet, and you look at who taught the computer to sing, or the Daisy Bell story, you’ll see Larry Kelly and Carol Lauchbaum mentioned and Carol Lauchbaum was Kelly’s Assistant, I’m sure she was a wonderful person.
But in truth, she was not the person who developed this work, it was Louis Gerstmann, Gerstmann and Kelly are painstakingly creating this model of the analog model of human speech. And they were working on this huge room sized IBM mainframe computer, they would have to input instructions very slowly. using punch cards, the computer would process the punch card instructions, and then it would output information onto a digital magnetic tape. But that magnetic tape would have to be running at an extremely slow speed because the processing power of that mainframe computer was paltry by today’s standards.
And then he would output this information to digital magnetic tape. And they would have to use this newly invented thing by Max Matthews called a digital to analog converter to convert the digits on the tape into sound that could be recorded onto an analog tape. And so every time that they changed the model of speech to try to tweak something, they would have to go through this long painstaking process to do so.
Ben Lindquist 39:00
Max Matthews or others who wrote about early computer music talk about this a lot, you know, the problem with the the slow feedback loop. So if I’m learning to play the violin, it might be a slow and arduous process. But as soon as I can hear the chord here was discordant or not discordant, and make the appropriate adjustments. But the problem that they had both with speech and music was that it would take a few days after they would hear whatever it was that they put the paper down in actual sound. And as a result, this is kind of used as the sort of excuse for why for example, the earliest instances of computer music the famous album music from mathematics at Bell Labs released in I think 1961 sounded so bad, right?
They said like Well, it’s interesting theoretically, and this is this is where the future lies they thought but for the moment because the feedback loop is so slow, and it makes it really really difficult to master this machine in the way that we can now master analog instrument that for now it’s More about the idea than it is about the actual sound. Yeah.
Mack Hagood 40:03
And so, you know, by the time in the 1960s, when they did create this recording of A Bicycle Built for Two, I mean, it’s hard to overstate what an accomplishment this was. Because not only did they have to get all the phonetics right, they also had to do pitch. And then Max Matthews creates the musical accompaniment over which the voices sing.
And all of that has to be temporally tight, right? Like the voice and the music have to happen together. And all of this, as you say, when there’s such a slow feedback loop, where you have to wait, literally like days in order to hear what you did. I mean, this must have been incredibly difficult, incredibly tedious. And it’s also I think, just for even for people who do know this history, I think for a lot of people, they get the idea that the computer was just singing, or the computer was just playing music in real time, it couldn’t be further from the case, the computer was very, very slowly putting out these musical notes, these individual bits of speech.
And a magnetic tape was running extremely slowly capturing these sounds, so that when you played it back at normal speed, suddenly you would have a performance that sounded like the computer was actually speaking in real time. And it was going to be many years before we could get into the era that we’re in now where computers actually could speak in real time. But that completely gets alighted, you know? So basically, what I’m saying is Kubrick heard a recording, he heard a recording of the Daisy Bell performance. He didn’t hear the computer do Daisy Bell, but in Yeah, in the cultural imagination, how is singing in real time? It would be decades before we could actually catch up to that.
Ben Lindquist 42:00
So while it kind of gave the impression of this sort of push button, automatic automated future, what was actually being heard was something that had been finished previously. So if Kubrick had said, Well, can you program this song? Have your IBM sing a rendition of my favorite Beatles tune? Or can you say “Hi, Stanley Kubrick? How are you today” that wouldn’t have been possible or it would have taken at least a few weeks before the computer could have responded to that input.
And so yeah, it was quite a difficult process. It was like the result of a few years of very hard work and 10 years prior work at Haskins lab, developing the rules that were used by Bell Labs.
Mack Hagood 42:44
And yet, despite all the decades of work, reducing human speech to its barest elements, trying to find the essential rules that would allow you to build up a new speaking voice from these bits and pieces. And despite the cultural influence that this method had, through HAL in 2001, A Space Odyssey, or even the prosthetic voice of Stephen Hawking, the phonemic approach of Louis Gerstmann turned out to be something of a dead end.
You see, it was quite capable of synthesizing an intelligible voice, but it would never be capable of creating a natural sounding voice, a voice that might someday pass a vocal Turing test.
Ben Lindquist 43:31
Eventually what happens is they conclude that using this kind of speech that’s sort of built from scratch built from like the phoneme up just never really, it didn’t sound it never sounded as natural or as intelligible as they wanted it to.
Yeah, so they started in the 1990s. They they moved over to this thing called diphone synthesis, where they would rebuild speech from tiny little adjacent half phonemes right so you don’t use phonemes but you use two half phonemes that’s the fundamental unit of speech and then using these you can recreate speech that sounds somewhat more natural, that sounds less computerized or like mechanical and this is the speech that Siri used. The problem with that is that to make sure that these little bits of speech fit together these voice actors who are used have to speak in a very sort of flat monotone emotionally impoverished tone. So eventually, like much more recently scientists have relied like everyone else on neural nets. These machines are this learning process that can create a much more complicated and intricate set of rules that rebuild speech
Mack Hagood 44:52
And scratch and like so many things, I believe, like we start off by saying, Okay, if we can just distill it into what the underlying set of rules are, then we can reproduce anything we want. But it seems like with so many things in the digital world, we eventually came to realize, well, if we could just amass a huge set of data, the computer itself can extrapolate a whole set of rules that we can’t even comprehend, but it’ll be able to reproduce the things we want. Am I kind of glossing over that? Correct?
Ben Lindquist 45:25
I think that I think that gets at it fairly well, essentially, the rules were just especially if you want, not if you want to make speech that’s intelligible because speech was rules written by humans can create perfectly and do create perfectly intelligible speech. So you could think of Stephen Hawking’s voice, which, which is the most famous, I’d say, like the text to speech system from the 1980s. It was created by some MIT professor named Dennis Klatt, and it’s quite intelligible. It’s very intelligible , but it doesn’t sound natural, right?
So the intricacy of the rules and the profundity of the linguistic knowledge required to write those rules is just beyond the power of linguists. Yeah, I think Do you know, the problem with rules, of course, is that you’re taking. When we think of speech, and we think of speech that’s natural, it’s, it sounds spontaneous, it’s surprising. It’s very dry. If it’s not like that, we can think of read speech as a semi kind of mechanical speech, which is difficult to listen to. It sounds almost machine like it’s much more rule bound actually, than spoken speech.
The problem with spoken speech is that since it’s not read, since it’s spontaneous, it’s much harder to study. Yeah, right. So like speech, scientists realized after a point that one of the problems one of the reasons their devices sounded so mechanical, was that their knowledge of speech was based on the study of read speech, and read speech, just in a way it’s, in some ways, it’s not even speech, it should be thought of, they can read as more text like, right, it’s built from, from the phoneme up. And as a result, that’s just it, it doesn’t sound like speech.
Mack Hagood 47:06
And these so-called rules of speech. They are, you know, it’s basically like, we want to treat it like it’s a platonic ideal, and that all real lives, natural speech is just extrapolated from that. But it’s, in fact, that’s not the case. These are just approximations of patterns that have been observed. And yet real life never conforms neatly to them.
It reminds me of the of the history of electronic music and synthesis, where people kind of extracted a set of rules about how sounds happen, okay, there’s, there’s a volume envelope, right, and there’s the sustain, there’s an attack, there’s a release, and you could construct a synthetic instrument that sounded a lot like a trumpet by following those so called rules of what trumpets do. But it never sounded really like a trumpet until people just said, Well, hey, why don’t we just sample the trumpet? We’ll take a recording of this on the trumpet. And then we’ll manipulate that recording and allow you to articulate what the trumpet does in a bunch of different ways, using the actual live sound of the trumpet rather than modeling the trumpet.
Ben Lindquist 48:23
I think that gets back to my big takeaway. And I think that’s my big takeaway, namely, that the problem with creating a system of rules for something like speech is the more rule bound spontaneous speech is, the less that actually sounds like speech.
So how do you create a set of rules for something that’s dynamic and fluid and unexpected and as rich and dynamic as speeches? And that’s like a really interesting and challenging problem. And that’s sort of the problem that I try to hash out in my forthcoming long, forthcoming, distantly forthcoming book.
Mack Hagood 49:00
Fantastic. Well, Ben, thank you so much for this conversation. I’ve really enjoyed it and best of luck going forward for you and working on that first book.
Ben Lindquist 49:14
Yeah, thanks. Thanks. Thanks for having me. This was a lot of fun.
Mack Hagood 49:26
And that’s it for this episode of phantom power. Huge thanks to Benjamin Lindquist. You can find more information on Ben and find the link to his new paper in Critical Inquiry on the Art of Text to Speech in the show notes or on our website at phantompod.org where you can find all of our past episodes and so much more.
And speaking of speaking, you can speak to me and to all of our listeners just go to speakpipe.com/phantom power and leave us a voice message. We’d love to hear from you. Today Show is edited by Nisso Sacha and me and our transcript and show page were by Katelyn Phan and our website SEO and social media by Devin Ankeney. I’ll talk to you again in two weeks. Bye
The post From HAL to SIRI: How Computers Learned to Speak (Benjamin Lindquist) appeared first on Phantom Power.